Question about point mass prior and continuous distribution
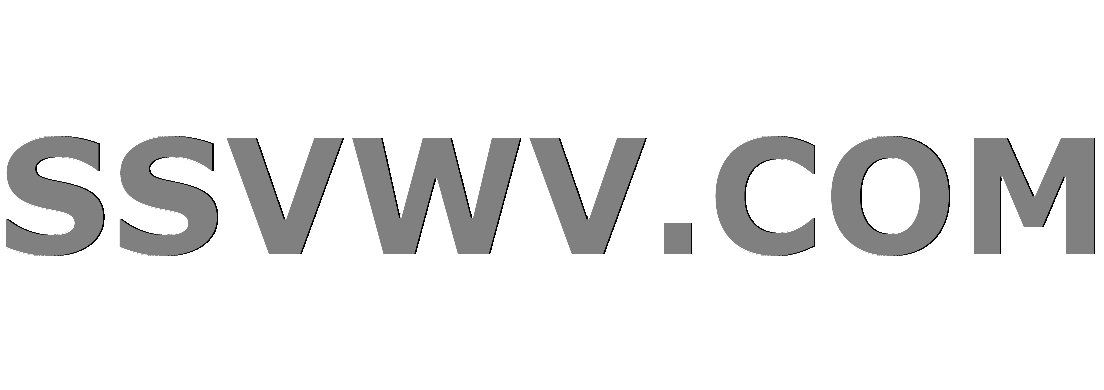
Multi tool use
.everyoneloves__top-leaderboard:empty,.everyoneloves__mid-leaderboard:empty{ margin-bottom:0;
}
up vote
1
down vote
favorite
Suppose we have a point mass prior,
$$theta sim begin{cases} I(theta=1) ,& prob=frac{1}{2} \
Gamma(c,c), & prob=frac{1}{2} end{cases}$$
Then if we are asked
$lim_{c to infty} P(theta=1)$
Now here is the issue, since Gamma is a continuous distribution, it seems that in the case of gamma,we will never have $theta=1$.
To me it thus seems that regardless of the value of c, the $p(theta=1)=frac{1}{2}$
However, we also have that since expected value of a $gamma(a,b)=frac{a}{b}$ so that the expected value of the gamma is 1 when we have $a=b=c$
But, by Markov, for $X sim Gamma(c,c)$
$lim_{c to infty} Pr(|X-mu| lt epsilon) to 1$ for any $epsilon gt 0$
So is $lim_{c to infty}P(theta=1) =1$ , or is $lim_{c to infty}P(theta=1)=frac{1}{2}$
As even though the markov inequality holds, it is a continous distirbution, so we will never have it exactly equal to 1.
Thanks all
bayesian continuous-data
add a comment |
up vote
1
down vote
favorite
Suppose we have a point mass prior,
$$theta sim begin{cases} I(theta=1) ,& prob=frac{1}{2} \
Gamma(c,c), & prob=frac{1}{2} end{cases}$$
Then if we are asked
$lim_{c to infty} P(theta=1)$
Now here is the issue, since Gamma is a continuous distribution, it seems that in the case of gamma,we will never have $theta=1$.
To me it thus seems that regardless of the value of c, the $p(theta=1)=frac{1}{2}$
However, we also have that since expected value of a $gamma(a,b)=frac{a}{b}$ so that the expected value of the gamma is 1 when we have $a=b=c$
But, by Markov, for $X sim Gamma(c,c)$
$lim_{c to infty} Pr(|X-mu| lt epsilon) to 1$ for any $epsilon gt 0$
So is $lim_{c to infty}P(theta=1) =1$ , or is $lim_{c to infty}P(theta=1)=frac{1}{2}$
As even though the markov inequality holds, it is a continous distirbution, so we will never have it exactly equal to 1.
Thanks all
bayesian continuous-data
add a comment |
up vote
1
down vote
favorite
up vote
1
down vote
favorite
Suppose we have a point mass prior,
$$theta sim begin{cases} I(theta=1) ,& prob=frac{1}{2} \
Gamma(c,c), & prob=frac{1}{2} end{cases}$$
Then if we are asked
$lim_{c to infty} P(theta=1)$
Now here is the issue, since Gamma is a continuous distribution, it seems that in the case of gamma,we will never have $theta=1$.
To me it thus seems that regardless of the value of c, the $p(theta=1)=frac{1}{2}$
However, we also have that since expected value of a $gamma(a,b)=frac{a}{b}$ so that the expected value of the gamma is 1 when we have $a=b=c$
But, by Markov, for $X sim Gamma(c,c)$
$lim_{c to infty} Pr(|X-mu| lt epsilon) to 1$ for any $epsilon gt 0$
So is $lim_{c to infty}P(theta=1) =1$ , or is $lim_{c to infty}P(theta=1)=frac{1}{2}$
As even though the markov inequality holds, it is a continous distirbution, so we will never have it exactly equal to 1.
Thanks all
bayesian continuous-data
Suppose we have a point mass prior,
$$theta sim begin{cases} I(theta=1) ,& prob=frac{1}{2} \
Gamma(c,c), & prob=frac{1}{2} end{cases}$$
Then if we are asked
$lim_{c to infty} P(theta=1)$
Now here is the issue, since Gamma is a continuous distribution, it seems that in the case of gamma,we will never have $theta=1$.
To me it thus seems that regardless of the value of c, the $p(theta=1)=frac{1}{2}$
However, we also have that since expected value of a $gamma(a,b)=frac{a}{b}$ so that the expected value of the gamma is 1 when we have $a=b=c$
But, by Markov, for $X sim Gamma(c,c)$
$lim_{c to infty} Pr(|X-mu| lt epsilon) to 1$ for any $epsilon gt 0$
So is $lim_{c to infty}P(theta=1) =1$ , or is $lim_{c to infty}P(theta=1)=frac{1}{2}$
As even though the markov inequality holds, it is a continous distirbution, so we will never have it exactly equal to 1.
Thanks all
bayesian continuous-data
bayesian continuous-data
edited 4 hours ago
asked 5 hours ago
Learning
418
418
add a comment |
add a comment |
1 Answer
1
active
oldest
votes
up vote
3
down vote
accepted
Your specified prior distribution is a mixture of a continuous and discrete part. Let $I sim text{Bern}(1/2)$ be the indicator that the parameter is taken from the gamma distribution. Then using the law of total probability you have:
$$begin{equation} begin{aligned}
mathbb{P}(theta = 1|c)
&= mathbb{P}(theta = 1|c, I=0) cdot mathbb{P}(I=0) + mathbb{P}(theta = 1|c, I=1) cdot mathbb{P}(I=1) \[6pt]
&= frac{1}{2} cdot mathbb{P}(theta = 1|c, I=0) + frac{1}{2} cdot mathbb{P}(theta = 1|c, I=1) \[6pt]
&= frac{1}{2} + frac{1}{2} cdot mathbb{P}(theta = 1| theta sim text{Ga}(c,c)) \[6pt]
&= frac{1}{2}. \[6pt]
end{aligned} end{equation}$$
(Note that the last step comes from recognising that the gamma is a continuous distribution, so the probability of a specific point is zero under this distribution.) This result holds for all values of $c$, so you are correct that it also holds in the limit:
$$lim_{c rightarrow infty} mathbb{P}(theta = 1|c) = lim_{c rightarrow infty} frac{1}{2} = frac{1}{2}.$$
Your later use of Chebychev's inequality shows that as $c rightarrow infty$ you get $theta rightarrow 1$ (convergence in probability), but this does not change the fact that $mathbb{P}(theta = 1|c) = 1/2$ for all $c > 0$. (To understand the reason for this more fully, have a read about the distinction between convergence in probability and almost-sure convergence.)
add a comment |
1 Answer
1
active
oldest
votes
1 Answer
1
active
oldest
votes
active
oldest
votes
active
oldest
votes
up vote
3
down vote
accepted
Your specified prior distribution is a mixture of a continuous and discrete part. Let $I sim text{Bern}(1/2)$ be the indicator that the parameter is taken from the gamma distribution. Then using the law of total probability you have:
$$begin{equation} begin{aligned}
mathbb{P}(theta = 1|c)
&= mathbb{P}(theta = 1|c, I=0) cdot mathbb{P}(I=0) + mathbb{P}(theta = 1|c, I=1) cdot mathbb{P}(I=1) \[6pt]
&= frac{1}{2} cdot mathbb{P}(theta = 1|c, I=0) + frac{1}{2} cdot mathbb{P}(theta = 1|c, I=1) \[6pt]
&= frac{1}{2} + frac{1}{2} cdot mathbb{P}(theta = 1| theta sim text{Ga}(c,c)) \[6pt]
&= frac{1}{2}. \[6pt]
end{aligned} end{equation}$$
(Note that the last step comes from recognising that the gamma is a continuous distribution, so the probability of a specific point is zero under this distribution.) This result holds for all values of $c$, so you are correct that it also holds in the limit:
$$lim_{c rightarrow infty} mathbb{P}(theta = 1|c) = lim_{c rightarrow infty} frac{1}{2} = frac{1}{2}.$$
Your later use of Chebychev's inequality shows that as $c rightarrow infty$ you get $theta rightarrow 1$ (convergence in probability), but this does not change the fact that $mathbb{P}(theta = 1|c) = 1/2$ for all $c > 0$. (To understand the reason for this more fully, have a read about the distinction between convergence in probability and almost-sure convergence.)
add a comment |
up vote
3
down vote
accepted
Your specified prior distribution is a mixture of a continuous and discrete part. Let $I sim text{Bern}(1/2)$ be the indicator that the parameter is taken from the gamma distribution. Then using the law of total probability you have:
$$begin{equation} begin{aligned}
mathbb{P}(theta = 1|c)
&= mathbb{P}(theta = 1|c, I=0) cdot mathbb{P}(I=0) + mathbb{P}(theta = 1|c, I=1) cdot mathbb{P}(I=1) \[6pt]
&= frac{1}{2} cdot mathbb{P}(theta = 1|c, I=0) + frac{1}{2} cdot mathbb{P}(theta = 1|c, I=1) \[6pt]
&= frac{1}{2} + frac{1}{2} cdot mathbb{P}(theta = 1| theta sim text{Ga}(c,c)) \[6pt]
&= frac{1}{2}. \[6pt]
end{aligned} end{equation}$$
(Note that the last step comes from recognising that the gamma is a continuous distribution, so the probability of a specific point is zero under this distribution.) This result holds for all values of $c$, so you are correct that it also holds in the limit:
$$lim_{c rightarrow infty} mathbb{P}(theta = 1|c) = lim_{c rightarrow infty} frac{1}{2} = frac{1}{2}.$$
Your later use of Chebychev's inequality shows that as $c rightarrow infty$ you get $theta rightarrow 1$ (convergence in probability), but this does not change the fact that $mathbb{P}(theta = 1|c) = 1/2$ for all $c > 0$. (To understand the reason for this more fully, have a read about the distinction between convergence in probability and almost-sure convergence.)
add a comment |
up vote
3
down vote
accepted
up vote
3
down vote
accepted
Your specified prior distribution is a mixture of a continuous and discrete part. Let $I sim text{Bern}(1/2)$ be the indicator that the parameter is taken from the gamma distribution. Then using the law of total probability you have:
$$begin{equation} begin{aligned}
mathbb{P}(theta = 1|c)
&= mathbb{P}(theta = 1|c, I=0) cdot mathbb{P}(I=0) + mathbb{P}(theta = 1|c, I=1) cdot mathbb{P}(I=1) \[6pt]
&= frac{1}{2} cdot mathbb{P}(theta = 1|c, I=0) + frac{1}{2} cdot mathbb{P}(theta = 1|c, I=1) \[6pt]
&= frac{1}{2} + frac{1}{2} cdot mathbb{P}(theta = 1| theta sim text{Ga}(c,c)) \[6pt]
&= frac{1}{2}. \[6pt]
end{aligned} end{equation}$$
(Note that the last step comes from recognising that the gamma is a continuous distribution, so the probability of a specific point is zero under this distribution.) This result holds for all values of $c$, so you are correct that it also holds in the limit:
$$lim_{c rightarrow infty} mathbb{P}(theta = 1|c) = lim_{c rightarrow infty} frac{1}{2} = frac{1}{2}.$$
Your later use of Chebychev's inequality shows that as $c rightarrow infty$ you get $theta rightarrow 1$ (convergence in probability), but this does not change the fact that $mathbb{P}(theta = 1|c) = 1/2$ for all $c > 0$. (To understand the reason for this more fully, have a read about the distinction between convergence in probability and almost-sure convergence.)
Your specified prior distribution is a mixture of a continuous and discrete part. Let $I sim text{Bern}(1/2)$ be the indicator that the parameter is taken from the gamma distribution. Then using the law of total probability you have:
$$begin{equation} begin{aligned}
mathbb{P}(theta = 1|c)
&= mathbb{P}(theta = 1|c, I=0) cdot mathbb{P}(I=0) + mathbb{P}(theta = 1|c, I=1) cdot mathbb{P}(I=1) \[6pt]
&= frac{1}{2} cdot mathbb{P}(theta = 1|c, I=0) + frac{1}{2} cdot mathbb{P}(theta = 1|c, I=1) \[6pt]
&= frac{1}{2} + frac{1}{2} cdot mathbb{P}(theta = 1| theta sim text{Ga}(c,c)) \[6pt]
&= frac{1}{2}. \[6pt]
end{aligned} end{equation}$$
(Note that the last step comes from recognising that the gamma is a continuous distribution, so the probability of a specific point is zero under this distribution.) This result holds for all values of $c$, so you are correct that it also holds in the limit:
$$lim_{c rightarrow infty} mathbb{P}(theta = 1|c) = lim_{c rightarrow infty} frac{1}{2} = frac{1}{2}.$$
Your later use of Chebychev's inequality shows that as $c rightarrow infty$ you get $theta rightarrow 1$ (convergence in probability), but this does not change the fact that $mathbb{P}(theta = 1|c) = 1/2$ for all $c > 0$. (To understand the reason for this more fully, have a read about the distinction between convergence in probability and almost-sure convergence.)
answered 4 hours ago


Ben
19.5k22295
19.5k22295
add a comment |
add a comment |
Thanks for contributing an answer to Cross Validated!
- Please be sure to answer the question. Provide details and share your research!
But avoid …
- Asking for help, clarification, or responding to other answers.
- Making statements based on opinion; back them up with references or personal experience.
Use MathJax to format equations. MathJax reference.
To learn more, see our tips on writing great answers.
Some of your past answers have not been well-received, and you're in danger of being blocked from answering.
Please pay close attention to the following guidance:
- Please be sure to answer the question. Provide details and share your research!
But avoid …
- Asking for help, clarification, or responding to other answers.
- Making statements based on opinion; back them up with references or personal experience.
To learn more, see our tips on writing great answers.
Sign up or log in
StackExchange.ready(function () {
StackExchange.helpers.onClickDraftSave('#login-link');
});
Sign up using Google
Sign up using Facebook
Sign up using Email and Password
Post as a guest
Required, but never shown
StackExchange.ready(
function () {
StackExchange.openid.initPostLogin('.new-post-login', 'https%3a%2f%2fstats.stackexchange.com%2fquestions%2f379550%2fquestion-about-point-mass-prior-and-continuous-distribution%23new-answer', 'question_page');
}
);
Post as a guest
Required, but never shown
Sign up or log in
StackExchange.ready(function () {
StackExchange.helpers.onClickDraftSave('#login-link');
});
Sign up using Google
Sign up using Facebook
Sign up using Email and Password
Post as a guest
Required, but never shown
Sign up or log in
StackExchange.ready(function () {
StackExchange.helpers.onClickDraftSave('#login-link');
});
Sign up using Google
Sign up using Facebook
Sign up using Email and Password
Post as a guest
Required, but never shown
Sign up or log in
StackExchange.ready(function () {
StackExchange.helpers.onClickDraftSave('#login-link');
});
Sign up using Google
Sign up using Facebook
Sign up using Email and Password
Sign up using Google
Sign up using Facebook
Sign up using Email and Password
Post as a guest
Required, but never shown
Required, but never shown
Required, but never shown
Required, but never shown
Required, but never shown
Required, but never shown
Required, but never shown
Required, but never shown
Required, but never shown
9sV axmq9DgajX5zQDyyRYZx1W90zPi68WdCOPTenI iqErinvUkq49Vgn H cVqh,Kb 49mqFzcRBL