The Book of Why by Judea Pearl: Why is he bashing statistics?
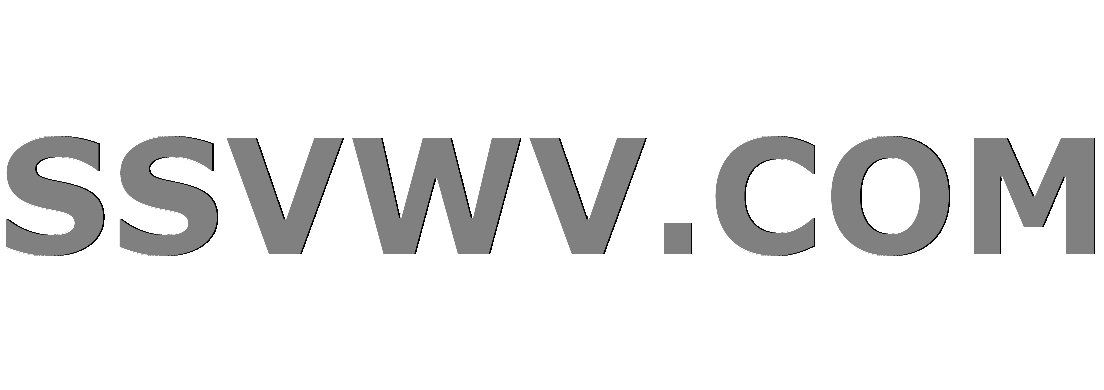
Multi tool use
.everyoneloves__top-leaderboard:empty,.everyoneloves__mid-leaderboard:empty{ margin-bottom:0;
}
up vote
62
down vote
favorite
I am reading The Book of Why by Judea Pearl, and it is getting under my skin1. Specifically, it appears to me that he is unconditionally bashing "classical" statistics by putting up a straw man argument that statistics is never, ever able to investigate causal relations, that it never is interested in causal relations, and that statistics "became a model-blinded data-reduction enterprise". Statistics becomes an ugly s-word in his book.
For example:
Statisticians have been immensely confused about what variables should and should not be controlled for, so the default practice has been to control for everything one can measure. [...] It is a convenient, simple procedure to follow, but it is both wasteful and ridden with errors. A key achievement of the Causal Revolution has been to bring an end to this confusion.
At the same time, statisticians greatly underrate controlling in the sense that they are loath to talk about causality at all [...]
However, causal models have been in statistics like, forever. I mean, a regression model can be used essentially a causal model, since we are essentially assuming that one variable is the cause and another is the effect (hence correlation is different approach from regression modelling) and testing whether this causal relationship explains the observed patterns.
The amount of misrepresentation of s-word which, as I perceive it, exists in this book made me wonder whether causal inference (which hitherto I perceived as a useful and interesting way of organizing and testing a scientific hypothesis2) is questionable.
Questions: do you think that Judea Pearl is misrepresenting statistics, and if yes, why? Just to make causal inference sound bigger than it is? Do you think that causal inference is a Revolution with a big R which really changes all our thinking?
1. Also because he is such a modest guy.
2. I mean in the scientific, not statistical sense.
causality
|
show 15 more comments
up vote
62
down vote
favorite
I am reading The Book of Why by Judea Pearl, and it is getting under my skin1. Specifically, it appears to me that he is unconditionally bashing "classical" statistics by putting up a straw man argument that statistics is never, ever able to investigate causal relations, that it never is interested in causal relations, and that statistics "became a model-blinded data-reduction enterprise". Statistics becomes an ugly s-word in his book.
For example:
Statisticians have been immensely confused about what variables should and should not be controlled for, so the default practice has been to control for everything one can measure. [...] It is a convenient, simple procedure to follow, but it is both wasteful and ridden with errors. A key achievement of the Causal Revolution has been to bring an end to this confusion.
At the same time, statisticians greatly underrate controlling in the sense that they are loath to talk about causality at all [...]
However, causal models have been in statistics like, forever. I mean, a regression model can be used essentially a causal model, since we are essentially assuming that one variable is the cause and another is the effect (hence correlation is different approach from regression modelling) and testing whether this causal relationship explains the observed patterns.
The amount of misrepresentation of s-word which, as I perceive it, exists in this book made me wonder whether causal inference (which hitherto I perceived as a useful and interesting way of organizing and testing a scientific hypothesis2) is questionable.
Questions: do you think that Judea Pearl is misrepresenting statistics, and if yes, why? Just to make causal inference sound bigger than it is? Do you think that causal inference is a Revolution with a big R which really changes all our thinking?
1. Also because he is such a modest guy.
2. I mean in the scientific, not statistical sense.
causality
35
Linear regression is not a causal model. Simple linear regression is the same as pairwise correlation, the only difference is standarization. So if you say that regression is causal, then same should be true also for correlation. Is correlation causation? You can use regression to predict whatever, nonsense relations between any arbitrary variables (with many "significant" results by chance).
– Tim♦
Nov 14 at 9:35
7
Disagreements over which approach to reasoning about causality in statistics has most merit between Pearl, Rubin, Heckman and others appear to have festered, and I do think Pearl's tone is getting ever haughtier. Don't let that distract you from the genuine insight he has to offer. Read his earlier book Causality, it will get under your skin less.
– CloseToC
Nov 14 at 10:01
6
@CloseToC I would add that Pearl, Rubin and Heckman are in a way all working within the same framework (i.e., logically equivalent frameworks, see here stats.stackexchange.com/questions/249767/…), so their disputes are in a different level from arguing things like "linear regression is a causal model".
– Carlos Cinelli
Nov 14 at 10:04
8
I have been irritated by the book myself. There are some simply false statistical claims there (cannot cite now, the book with my notes in the margins is at home) which made me wonder whether only the journalist who helped Pearl write the book or also Pearl himself was a poor statistician. (Needless to say, I was very surprised to discover such blatant mistakes in a work of such a revered scientist.) His papers are much better, though even there no one would accuse Pearl for modesty...
– Richard Hardy
Nov 14 at 13:32
14
I have some concern that this thread already tangles together (a) a specific book from a very smart person (b) that smart person's persona and style of debate (c) whether a particular viewpoint is correct, exaggerated, or whatever.
– Nick Cox
Nov 14 at 13:41
|
show 15 more comments
up vote
62
down vote
favorite
up vote
62
down vote
favorite
I am reading The Book of Why by Judea Pearl, and it is getting under my skin1. Specifically, it appears to me that he is unconditionally bashing "classical" statistics by putting up a straw man argument that statistics is never, ever able to investigate causal relations, that it never is interested in causal relations, and that statistics "became a model-blinded data-reduction enterprise". Statistics becomes an ugly s-word in his book.
For example:
Statisticians have been immensely confused about what variables should and should not be controlled for, so the default practice has been to control for everything one can measure. [...] It is a convenient, simple procedure to follow, but it is both wasteful and ridden with errors. A key achievement of the Causal Revolution has been to bring an end to this confusion.
At the same time, statisticians greatly underrate controlling in the sense that they are loath to talk about causality at all [...]
However, causal models have been in statistics like, forever. I mean, a regression model can be used essentially a causal model, since we are essentially assuming that one variable is the cause and another is the effect (hence correlation is different approach from regression modelling) and testing whether this causal relationship explains the observed patterns.
The amount of misrepresentation of s-word which, as I perceive it, exists in this book made me wonder whether causal inference (which hitherto I perceived as a useful and interesting way of organizing and testing a scientific hypothesis2) is questionable.
Questions: do you think that Judea Pearl is misrepresenting statistics, and if yes, why? Just to make causal inference sound bigger than it is? Do you think that causal inference is a Revolution with a big R which really changes all our thinking?
1. Also because he is such a modest guy.
2. I mean in the scientific, not statistical sense.
causality
I am reading The Book of Why by Judea Pearl, and it is getting under my skin1. Specifically, it appears to me that he is unconditionally bashing "classical" statistics by putting up a straw man argument that statistics is never, ever able to investigate causal relations, that it never is interested in causal relations, and that statistics "became a model-blinded data-reduction enterprise". Statistics becomes an ugly s-word in his book.
For example:
Statisticians have been immensely confused about what variables should and should not be controlled for, so the default practice has been to control for everything one can measure. [...] It is a convenient, simple procedure to follow, but it is both wasteful and ridden with errors. A key achievement of the Causal Revolution has been to bring an end to this confusion.
At the same time, statisticians greatly underrate controlling in the sense that they are loath to talk about causality at all [...]
However, causal models have been in statistics like, forever. I mean, a regression model can be used essentially a causal model, since we are essentially assuming that one variable is the cause and another is the effect (hence correlation is different approach from regression modelling) and testing whether this causal relationship explains the observed patterns.
The amount of misrepresentation of s-word which, as I perceive it, exists in this book made me wonder whether causal inference (which hitherto I perceived as a useful and interesting way of organizing and testing a scientific hypothesis2) is questionable.
Questions: do you think that Judea Pearl is misrepresenting statistics, and if yes, why? Just to make causal inference sound bigger than it is? Do you think that causal inference is a Revolution with a big R which really changes all our thinking?
1. Also because he is such a modest guy.
2. I mean in the scientific, not statistical sense.
causality
causality
edited Nov 14 at 16:43
gung♦
105k34253515
105k34253515
asked Nov 14 at 9:22
January
4,3742248
4,3742248
35
Linear regression is not a causal model. Simple linear regression is the same as pairwise correlation, the only difference is standarization. So if you say that regression is causal, then same should be true also for correlation. Is correlation causation? You can use regression to predict whatever, nonsense relations between any arbitrary variables (with many "significant" results by chance).
– Tim♦
Nov 14 at 9:35
7
Disagreements over which approach to reasoning about causality in statistics has most merit between Pearl, Rubin, Heckman and others appear to have festered, and I do think Pearl's tone is getting ever haughtier. Don't let that distract you from the genuine insight he has to offer. Read his earlier book Causality, it will get under your skin less.
– CloseToC
Nov 14 at 10:01
6
@CloseToC I would add that Pearl, Rubin and Heckman are in a way all working within the same framework (i.e., logically equivalent frameworks, see here stats.stackexchange.com/questions/249767/…), so their disputes are in a different level from arguing things like "linear regression is a causal model".
– Carlos Cinelli
Nov 14 at 10:04
8
I have been irritated by the book myself. There are some simply false statistical claims there (cannot cite now, the book with my notes in the margins is at home) which made me wonder whether only the journalist who helped Pearl write the book or also Pearl himself was a poor statistician. (Needless to say, I was very surprised to discover such blatant mistakes in a work of such a revered scientist.) His papers are much better, though even there no one would accuse Pearl for modesty...
– Richard Hardy
Nov 14 at 13:32
14
I have some concern that this thread already tangles together (a) a specific book from a very smart person (b) that smart person's persona and style of debate (c) whether a particular viewpoint is correct, exaggerated, or whatever.
– Nick Cox
Nov 14 at 13:41
|
show 15 more comments
35
Linear regression is not a causal model. Simple linear regression is the same as pairwise correlation, the only difference is standarization. So if you say that regression is causal, then same should be true also for correlation. Is correlation causation? You can use regression to predict whatever, nonsense relations between any arbitrary variables (with many "significant" results by chance).
– Tim♦
Nov 14 at 9:35
7
Disagreements over which approach to reasoning about causality in statistics has most merit between Pearl, Rubin, Heckman and others appear to have festered, and I do think Pearl's tone is getting ever haughtier. Don't let that distract you from the genuine insight he has to offer. Read his earlier book Causality, it will get under your skin less.
– CloseToC
Nov 14 at 10:01
6
@CloseToC I would add that Pearl, Rubin and Heckman are in a way all working within the same framework (i.e., logically equivalent frameworks, see here stats.stackexchange.com/questions/249767/…), so their disputes are in a different level from arguing things like "linear regression is a causal model".
– Carlos Cinelli
Nov 14 at 10:04
8
I have been irritated by the book myself. There are some simply false statistical claims there (cannot cite now, the book with my notes in the margins is at home) which made me wonder whether only the journalist who helped Pearl write the book or also Pearl himself was a poor statistician. (Needless to say, I was very surprised to discover such blatant mistakes in a work of such a revered scientist.) His papers are much better, though even there no one would accuse Pearl for modesty...
– Richard Hardy
Nov 14 at 13:32
14
I have some concern that this thread already tangles together (a) a specific book from a very smart person (b) that smart person's persona and style of debate (c) whether a particular viewpoint is correct, exaggerated, or whatever.
– Nick Cox
Nov 14 at 13:41
35
35
Linear regression is not a causal model. Simple linear regression is the same as pairwise correlation, the only difference is standarization. So if you say that regression is causal, then same should be true also for correlation. Is correlation causation? You can use regression to predict whatever, nonsense relations between any arbitrary variables (with many "significant" results by chance).
– Tim♦
Nov 14 at 9:35
Linear regression is not a causal model. Simple linear regression is the same as pairwise correlation, the only difference is standarization. So if you say that regression is causal, then same should be true also for correlation. Is correlation causation? You can use regression to predict whatever, nonsense relations between any arbitrary variables (with many "significant" results by chance).
– Tim♦
Nov 14 at 9:35
7
7
Disagreements over which approach to reasoning about causality in statistics has most merit between Pearl, Rubin, Heckman and others appear to have festered, and I do think Pearl's tone is getting ever haughtier. Don't let that distract you from the genuine insight he has to offer. Read his earlier book Causality, it will get under your skin less.
– CloseToC
Nov 14 at 10:01
Disagreements over which approach to reasoning about causality in statistics has most merit between Pearl, Rubin, Heckman and others appear to have festered, and I do think Pearl's tone is getting ever haughtier. Don't let that distract you from the genuine insight he has to offer. Read his earlier book Causality, it will get under your skin less.
– CloseToC
Nov 14 at 10:01
6
6
@CloseToC I would add that Pearl, Rubin and Heckman are in a way all working within the same framework (i.e., logically equivalent frameworks, see here stats.stackexchange.com/questions/249767/…), so their disputes are in a different level from arguing things like "linear regression is a causal model".
– Carlos Cinelli
Nov 14 at 10:04
@CloseToC I would add that Pearl, Rubin and Heckman are in a way all working within the same framework (i.e., logically equivalent frameworks, see here stats.stackexchange.com/questions/249767/…), so their disputes are in a different level from arguing things like "linear regression is a causal model".
– Carlos Cinelli
Nov 14 at 10:04
8
8
I have been irritated by the book myself. There are some simply false statistical claims there (cannot cite now, the book with my notes in the margins is at home) which made me wonder whether only the journalist who helped Pearl write the book or also Pearl himself was a poor statistician. (Needless to say, I was very surprised to discover such blatant mistakes in a work of such a revered scientist.) His papers are much better, though even there no one would accuse Pearl for modesty...
– Richard Hardy
Nov 14 at 13:32
I have been irritated by the book myself. There are some simply false statistical claims there (cannot cite now, the book with my notes in the margins is at home) which made me wonder whether only the journalist who helped Pearl write the book or also Pearl himself was a poor statistician. (Needless to say, I was very surprised to discover such blatant mistakes in a work of such a revered scientist.) His papers are much better, though even there no one would accuse Pearl for modesty...
– Richard Hardy
Nov 14 at 13:32
14
14
I have some concern that this thread already tangles together (a) a specific book from a very smart person (b) that smart person's persona and style of debate (c) whether a particular viewpoint is correct, exaggerated, or whatever.
– Nick Cox
Nov 14 at 13:41
I have some concern that this thread already tangles together (a) a specific book from a very smart person (b) that smart person's persona and style of debate (c) whether a particular viewpoint is correct, exaggerated, or whatever.
– Nick Cox
Nov 14 at 13:41
|
show 15 more comments
7 Answers
7
active
oldest
votes
up vote
41
down vote
accepted
I fully agree that Pearl's tone is arrogant, and his characterisation of "statisticians" is simplistic and monolithic. Also, I don't find his writing particularly clear.
However, I think he has a point.
Causal reasoning was not part of my formal training (MSc): the closest I got to the topic was an elective course in experimental design, i.e. any causality claims required me to physically control the environment. Pearl's book Causality was my first exposure to a refutation of this idea. Obviously I can't speak for all statisticians and curricula, but from my own perspective I subscribe to Pearl's observation that causal reasoning is not a priority in statistics.
It is true that statisticians sometimes control for more variables than is strictly necessary, but this rarely leads to error (at least in my experience).
This is also a belief that I held after graduating with an MSc in statistics in 2010.
However, it is deeply incorrect. When you control for a common effect (called "collider" in the book), you can introduce selection bias. This realization was quite astonishing to me, and really convinced me of the usefulness of representing my causal hypotheses as graphs.
EDIT: I was asked to elaborate on selection bias. This topic is quite subtle, I highly recommend perusing the edX MOOC on Causal Diagrams, a very nice introduction to graphs which has a chapter dedicated to selection bias.
For a toy example, to paraphrase this paper cited in the book: Consider the variables A=attractiveness, B=beauty, C=competence. Suppose that B and C are causally unrelated in the general population (i.e., beauty does not cause competence, competence does not cause beauty, and beauty and competence do not share a common cause). Suppose also that any one of B or C is sufficient for being attractive, i.e. A is a collider. Conditioning on A creates a spurious association between B and C.
A more serious example is the "birth weight paradox", according to which a mother's smoking (S) during pregnancy seems to decrease the mortality (M) of the baby, if the baby is underweight (U). The proposed explanation is that birth defects (D) also cause low birth weight, and also contribute to mortality. The corresponding causal diagram is { S -> U, D -> U, U -> M, S -> M, D -> M } in which U is a collider; conditioning on it introduces the spurious association. The intuition behind this is that if the mother is a smoker, the low birth weight is less likely to be due to a defect.
7
+1. Can you elaborate just a little bit more on how it introduces selection bias? Perhaps a little concrete example will make it clear for most readers.
– amoeba
Nov 15 at 13:57
2
Thanks for the edit. These are very clear examples.
– amoeba
Nov 15 at 23:11
Regarding B and C are unassociated, aren't you misusing the language here? See Section 2.2 on p. 105 in Pearl "Statistics and Causal Inference: A Review" (2003) on language of association vs. causation.
– Richard Hardy
yesterday
1
@RichardHardy : good point -- fixed!
– mitchus
yesterday
add a comment |
up vote
57
down vote
Your very question reflects what Pearl is saying!
a simple linear regression is essentially a causal model
No, a linear regression is a statistical model, not a causal model. Let's assume $Y, X, Z$ are random variables with a multivariate normal distribution. Then you can correctly estimate the linear expectations $E[Ymid X]$, $E[Xmid Y]$, $E[Ymid X,Z]$, $E[Zmid Y, X]$ etc using linear regression, but there's nothing here that says whether any of those quantities are causal.
A linear structural equation, on the other hand, is a causal model. But the first step is to understand the difference between statistical assumptions (constraints on the observed joint probability distribution) and causal assumptions (constraints on the causal model).
do you think that Judea Pearl misrepresenting statistics, and if yes,
why?
No, I don't think so, because we see these misconceptions daily. Of course, Pearl is making some generalizations, since some statisticians do work with causal inference (Don Rubin was a pioneer in promoting potential outcomes... also, I am a statistician!). But he is correct in saying that the bulk of traditional statistics education shuns causality, even to formally define what a causal effect is.
To make this clear, if we ask a statistician/econometrician with just a regular training to define mathematically what is the expected value of $Y$ if we intervene on $X$, he would probably write $E[Y|X]$ (see an example here)! But that's an observational quantity, that's not how you define a causal effect! In other terms, currently, a student with just a traditional statistics course lacks even the ability of properly defining this quantity mathematically ($E[Y_{x}]$ or $E[Y|do(x)]$) if you are not familiar with the structural/counterfactual theory of causation!
The quote you bring from the book is also a great example. You will not find in traditional statistics books a correct definition of what a confounder is, nor guidance about when you should (or should not) adjust for a covariate in observational studies. In general, you see “correlational criteria”, such as “if the covariate is associated with the treatment and with the outcome, you should adjust for it”. One of the most notable examples of this confusion shows up in Simpson’s Paradox—when faced with two estimates of opposite signs, which one should you use, the adjusted or unadjusted? The, answer, of course, depends on the causal model.
And what does Pearl mean when he says that this question was brought to an end? In the case of simple adjustment via regression, he is referring to the backdoor criterion (see more here). And for identification in general---beyond simple adjustment---he means that we now have complete algorithms for identification of causal effects for any given semi-markovian DAG.
Another remark here is worth making. Even in experimental studies — where traditional statistics has surely done a lot of important work with the design of experiments!— in the end of the day you still need a causal model. Experiments can suffer from lack of compliance, from loss of follow up, from selection bias... also, most of the time you don't want to confine the results of your experiments to the specific population you analyzed, you want to generalize your experimental results to a broader/different population. Here, again, one may ask: what should you adjust for? Are the data and substantive knowledge you have enough to allow such extrapolation? All of these are causal concepts, thus you need a language to formally express causal assumptions and check whether they are enough to allow you to do what you want!
In sum, these misconceptions are widespread in statistics and econometrics, there are several examples here in Cross Validated, such as:
- understanding what a confounder is
- understanding which variables you should include in a regression (for estimating causal effects)
understanding that even if you include all variables a regression may not be causal- understanding the difference between (lack of) statistical associations and causation
- defining a causal model and causal effects
And many more.
Do you think that causal inference is a Revolution with a big R which
really changes all our thinking?
Considering the current state of affairs in many sciences, how much we have advanced and how fast things are changing, and how much we can still do, I would say this is indeed a revolution.
2
Thank you. But – well, writing simplistically, I can calculate E[X|Y] as well as E[Y|X], but I can write X←Y as well as X→Y in a DAG. One way or other, I must start with a scientific hypothesis or a model. My hypothesis, my model – my choice. The very fact that I can do something doesn't mean I should do it, does it.
– January
Nov 14 at 10:28
2
@January it doesn't mean you should, the point here is only about being able to articulate accurately what you want to estimate (the causal estimand), articulate accurately your causal assumptions (making clear the distinction of causal and statistical assumptions), checking the logical implications of those causal assumptions and being able to understand whether your causal assumptions + data are enough for answering your query.
– Carlos Cinelli
Nov 14 at 10:30
3
@January say you have an observational study and want to estimate the causal effect of $X$ on $Y$. How do you decide which covariates to include in your regression?
– Carlos Cinelli
Nov 14 at 10:37
4
I think so: it doesn't seem entirely unfair to suggest that your average statistician, while likely well versed in causal inference from controlled experiments, & certainly in no danger of confusing correlation with causation, might be a bit shaky on causal inference from observational data. I take the last to be the context of the quotation (I haven't read the book) & it's something that some readers of this post may not pick up on.
– Scortchi♦
Nov 14 at 17:15
4
@January In short "adjusting for covariates" does not necessarily mean you have eliminated bias in causal effect estimates from those variables.
– Alexis
Nov 14 at 22:50
|
show 13 more comments
up vote
24
down vote
I'm a fan of Judea's writing, and I've read Causality (love) and Book of Why (like).
I do not feel that Judea is bashing statistics. It's hard to hear criticism. But what can we say about any person or field that doesn't take criticism? They tend from greatness to complacency. You must ask: is the criticism correct, needed, useful, and does it propose alternatives? The answer to all those is an emphatic "Yes".
Correct? I've reviewed and collaborated on a few dozen papers, mostly analyses of observational data, and I rarely feel there is a sufficient discussion of causality. The "adjustment" approach involves selecting variables because they were hand-picked from the DD as being "useful" "relevant" "important" or other nonsense.$^1$
Needed? The media is awash with seemingly contradictory statements about the health effects of major exposures. Inconsistency with data analysis has stagnated evidence which leaves us lacking useful policy, healthcare procedures, and recommendations for better living.
Useful? Judea's comment is pertinent and specific enough to give pause. It is directly relevant to any data analysis any statistician or data expert might encounter.
Does it propose alternatives? Yes, Judea in fact discusses the possibility of advanced statistical methods, and even how they reduce to known statistical frameworks (like Structural Equation Modeling) and their connection to regression models). It all boils down to requiring an explicit statement of the content knowledge that has guided the modeling approach.
Judea isn't simply suggesting we defenestrate all statistical methods (e.g. regression). Rather, he is saying that we need to embrace some causal theory to justify models.
$^1$ the complaint here is about the use of convincing and imprecise language to justify what is ultimately the wrong approach to modeling. There can be overlap, serendipitously, but Pearl is clear about the purpose of a causal diagram (DAG) and how variables can be classified as "confounders".
3
Nice answer. Note that not being a statistician but having served as an interface between statistics and biology for many years, for me any criticism of statisticians is really not so hard to hear ;-) However, do you really think that "orthodox statistics" cannot handle causality at all, as Pearl explicitly states?
– January
Nov 14 at 15:11
3
@January au contraire. I think that the deficiency among statisticians in accepting the causal inference in their analyses is directly related to their deficiency in understanding frequentist inference. It's the counterfactual reasoning that lacks.
– AdamO
Nov 14 at 15:38
4
+1 "The "adjustment" approach involves selecting variables because they were hand-picked from the DD as being "useful" "relevant" "important" or other nonsense without actually incorporating formal hypotheses about the specific causal relationships among them (a la the formal use of DAGs) ." Edit added. :)
– Alexis
Nov 14 at 21:15
Comments are not for extended discussion; this conversation has been moved to chat.
– Scortchi♦
Nov 15 at 23:17
add a comment |
up vote
19
down vote
I haven't read this book, so I can only judge the particular quote you give. However, even on this basis, I agree with you that this seems extremely unfair to the statistical profession. I actually think that statisticians have always done a remarkably good job at stressing the distinction between statistical associations (correlation, etc.) and causality, and warning against the conflation of the two. Indeed, in my experience, statisticians have generally been the primary professional force fighting against the ubiquitous confusion between cause and correlation. It is outright false (and virtually slander) to claim that statisticians are "...loath to talk about causality at all." I can see why you are annoyed reading arrogant horseshit like this.
I would say that it is reasonably common for non-statisticians who use statistical models to have a poor understanding of the relationship between statistical association and causality. Some have good scientific training from other fields, in which case they may also be well aware of the issue, but there are certainly some people who use statistical models who have a poor grasp of these issues. This is true in many applied scientific fields where practitioners have basic training in statistics, but do not learn it at a deep level. In these cases it is often professional statisticians who alert other researchers to the distinctions between these concepts and their proper relationship. Statisticians are often the key designers of RCTs and other experiments involving controls used to isolate causality. They are often called on to explain protocols such as randomisation, placebos, and other protocols that are used to try to sever relationships with potential confounding variables. It is true that statisticians sometimes control for more variables than is strictly necessary, but this rarely leads to error (at least in my experience).
I think Judea Pearl has made a very valuable contribution to statistics with his work on causality, and I am grateful to him for this wonderful contribution. He has constructed and examined some very useful formalisms that help to isolate causal relationships, and his work has become a staple of a good statistical education. I read his book Causality while I was a grad student, and it is on my shelf, and on the shelves of many other statisticians. Much of this formalism echoes things that have been known intuitively to statisticians since before they were formalised into an algebraic system, but it is very valuable in any case, and goes beyond that which is obvious. (I actually think in the future we will see a merging of the "do" operation with probability algebra occurring at an axiomatic level, and this will probably eventually become the core of probability theory. I would love to see this built directly into statistical education, so that you learn about causal models and the "do" operation when you learn about probability measures.)
One final thing to bear in mind here is that there are many applications of statistics where the goal is predictive, where the practitioner is not seeking to infer causality. These types of applications are extremely common in statistics, and in such cases, it is important not to restrict oneself to causal relationships. This is true in most applications of statistics in finance, HR, workforce modelling, and many other fields. One should not underestimate the amount of contexts where one cannot or should not seek to control variables.
Update: I notice that my answer disagrees with the one provided by Carlos. Perhaps we disagree on what constitutes "a statistician/econometrician with just a regular training". Anyone who I would call a "statistician" usually has at least a graduate-level education, and usually has substantial professional training/experience. (For example, in Australia, the requirement to become an "Accredited Statistician" with our national professional body requires a minimum of four years experience after an honours degree, or six years experience after a regular bachelors degree.) In any case, a student studying statistics is not a statistician.
I notice that as evidence of the alleged lack of understanding of causality by statisticians, Carlos's answer points to several questions on CV.SE which ask about causality in regression. In every one of these cases, the question is asked by someone who is obviously a novice (not a statistician) and the answers given by Carlos and others (which reflect the correct explanation) are highly-upvoted answers. Indeed, in several of the cases Carlos has given a detailed account of the causality and his answers are the most highly up-voted. This surely proves that statisticians do understand causality.
12
I am a non-statistician whose formal training in statistics was by non-statisticians in the same field, and I teach and research with non-statisticians applying statistics. I can assure you that the principle that (e.g.) correlation is not causation is, and was, a recurrent mantra in my field. Indeed I don't come across people who can't see that a correlation between rainfall and wheat yield isn't all that needs to be said about the relationship between them and the underlying processes. Typically, in my experience, non-statisticians too have thought this through long since.
– Nick Cox
Nov 14 at 12:49
7
As an epidemiologist, I'm getting more and more annoyed by this mantra. As @NickCox says, this is something that even non-scientists understand. The problem I have is when everyone jumps on the bandwagon of "correlation does not mean causation!" whenever an observational study (a case-control study, say) is published. Yes, correlation does not mean causation but the researchers are usually quite aware of that and will do everything to design and analyze a study in such a way that a causal interpretation is at least plausible.
– COOLSerdash
Nov 14 at 12:59
4
@Nick Cox: I have edited to more accurately state that there are many non-statisticians who understand this well. It was not my intention to cast dispersions over other professions - only to stress that the issue is extremely well understood by statisticians.
– Ben
Nov 14 at 13:00
7
@NickCox There's a lot more to Pearl's contributions about causality than "correlation is not causation". I'm with Carlos here. There's enough to learn about causality that it should be a whole course. As far as I know, most statistics departments don't offer such a course.
– Neil G
Nov 15 at 0:35
11
@Ben : Pearl does not accuse statisticians of confusing correlation and causation. He accuses them of mostly steering clear of causal reasoning. I agree with you that his tone is arrogant, but I think he has a point.
– mitchus
Nov 15 at 13:19
|
show 9 more comments
up vote
8
down vote
a simple linear regression is essentially a causal model
Here's an example I came up with where a linear regression model fails to be causal. Let's say a priori that a drug was taken at time 0 (t=0) and that it has no effect on the rate of heart attacks at t=1. Heart attacks at t=1 affect heart attacks at t=2 (i.e. previous damage makes the heart more susceptible to damage). Survival at t=3 only depends on whether or not people had a heart attack at t=2 -- heart attack at t=1 realistically would affect survival at t=3, but we won't have an arrow, for the sake of simplicity.
Here's the legend:
Here's the true causal graph:
Let's pretend that we don't know that heart attacks at t=1 are independent of taking the drug at t=0 so we construct a simple linear regression model to estimate the effect of the drug on heart attack at t=0. Here our predictor would be Drug t=0 and our outcome variable would be Heart Attack t=1. The only data we have is people who survive at t=3, so we'll run our regression on that data.
Here's the 95% Bayesian credible interval for coefficient of Drug t=0:
Much of the probability as we can see is greater than 0, so it looks like there's an effect! However, we know a priori that there is 0 effect. The mathematics of causation as developed by Judea Pearl and others make it much easier to see that there will be bias in this example (due to conditioning on a descendant of a collider). Judea's work implies that in this situation, we should use the full data set (i.e. don't look at the people who only survived), which will remove the biased paths:
Here's the 95% Credible Interval when looking at the full data set (i.e. not conditioning on those who survived).
.
It is densely centered at 0, which essentially shows no association at all.
In real-life examples, things might not be so simple. There may be many more variables that might cause systematic bias (confounding, selection bias, etc.). What to adjust for in analyses has been mathematized by Pearl; algorithms can suggest which variable to adjust for, or even tell us when adjusting is not enough to remove systematic bias. With this formal theory set in place, we don't need to spend so much time arguing about what to adjust for and what not to adjust for; we can quickly reach conclusions as to whether or not our results are sound. We can design our experiments better, we can analyze observational data more easily.
Here's a freely-available course online on Causal DAGs by Miguel Hernàn. It has a bunch of real-life case studies where professors / scientists / statisticians have come to opposite conclusions about the question at hand. Some of them might seem like paradoxes. However, you can easily solve them via Judea Pearl's d-separation and backdoor-criterion.
For reference, here's code to the data-generating process and the code for credible intervals shown above:
import numpy as np
import pandas as pd
import statsmodels as sm
import pymc3 as pm
from sklearn.linear_model import LinearRegression
%matplotlib inline
# notice that taking the drug is independent of heart attack at time 1.
# heart_attack_time_1 doesn't "listen" to take_drug_t_0
take_drug_t_0 = np.random.binomial(n=1, p=0.7, size=10000)
heart_attack_time_1 = np.random.binomial(n=1, p=0.4, size=10000)
proba_heart_attack_time_2 =
# heart_attack_time_1 increases the probability of heart_attack_time_2. Let's say
# it's because it weakens the heart and makes it more susceptible to further
# injuries
#
# Yet, take_drug_t_0 decreases the probability of heart attacks happening at
# time 2
for drug_t_0, heart_attack_t_1 in zip(take_drug_t_0, heart_attack_time_1):
if drug_t_0 == 0 and heart_attack_t_1 == 0:
proba_heart_attack_time_2.append(0.1)
elif drug_t_0 == 1 and heart_attack_t_1 == 0:
proba_heart_attack_time_2.append(0.1)
elif drug_t_0 == 0 and heart_attack_t_1 == 1:
proba_heart_attack_time_2.append(0.5)
elif drug_t_0 == 1 and heart_attack_t_1 == 1:
proba_heart_attack_time_2.append(0.05)
heart_attack_time_2 = np.random.binomial(
n=2, p=proba_heart_attack_time_2, size=10000
)
# people who've had a heart attack at time 2 are more likely to die by time 3
proba_survive_t_3 =
for heart_attack_t_2 in heart_attack_time_2:
if heart_attack_t_2 == 0:
proba_survive_t_3.append(0.95)
else:
proba_survive_t_3.append(0.6)
survive_t_3 = np.random.binomial(
n=1, p=proba_survive_t_3, size=10000
)
df = pd.DataFrame(
{
'survive_t_3': survive_t_3,
'take_drug_t_0': take_drug_t_0,
'heart_attack_time_1': heart_attack_time_1,
'heart_attack_time_2': heart_attack_time_2
}
)
# we only have access to data of the people who survived
survive_t_3_data = df[
df['survive_t_3'] == 1
]
survive_t_3_X = survive_t_3_data[['take_drug_t_0']]
lr = LinearRegression()
lr.fit(survive_t_3_X, survive_t_3_data['heart_attack_time_1'])
lr.coef_
with pm.Model() as collider_bias_model_normal:
alpha = pm.Normal(name='alpha', mu=0, sd=1)
take_drug_t_0 = pm.Normal(name='take_drug_t_0', mu=0, sd=1)
summation = alpha + take_drug_t_0 * survive_t_3_data['take_drug_t_0']
sigma = pm.Exponential('sigma', lam=1)
pm.Normal(
name='observed',
mu=summation,
sd=sigma,
observed=survive_t_3_data['heart_attack_time_1']
)
collider_bias_normal_trace = pm.sample(2000, tune=1000)
pm.plot_posterior(collider_bias_normal_trace['take_drug_t_0'])
with pm.Model() as no_collider_bias_model_normal:
alpha = pm.Normal(name='alpha', mu=0, sd=1)
take_drug_t_0 = pm.Normal(name='take_drug_t_0', mu=0, sd=1)
summation = alpha + take_drug_t_0 * df['take_drug_t_0']
sigma = pm.Exponential('sigma', lam=1)
pm.Normal(
name='observed',
mu=summation,
sd=sigma,
observed=df['heart_attack_time_1']
)
no_collider_bias_normal_trace = pm.sample(2000, tune=2000)
pm.plot_posterior(no_collider_bias_normal_trace['take_drug_t_0'])
New contributor
edderic is a new contributor to this site. Take care in asking for clarification, commenting, and answering.
Check out our Code of Conduct.
add a comment |
up vote
4
down vote
Two papers, the second a classic, that help (I think) shed additional lights on Judea's points and this topic more generally. This comes from someone who has used SEM (which is correlation and regression) repeatedly and resonates with his critiques:
https://www.sciencedirect.com/science/article/pii/S0022103111001466
http://psycnet.apa.org/record/1973-20037-001
Essentially the papers describe why correlational models (regression) can not ordinarily be taken as implying any strong causal inference. Any pattern of associations can fit a given covariance matrix (i.e., non specification of direction and or relationship among the variables). Hence the need for such things as an experimental design, counterfactual propositions, etc. This even applies when one has a temporal structure to their data where the putative cause occurs in time before the putative effect.
add a comment |
up vote
1
down vote
"...since we are essentially assuming that one variable is the cause and another is the effect (hence correlation is different approach from regression modelling)..."
Regression modeling most definitely does NOT make this assumption.
"... and testing whether this causal relationship explains the observed patterns."
If you are assuming causality and validating it against observations, your are doing SEM modeling, or what Pearl would call SCM modeling. Whether or not you want to call that part of the domain of stats is debatable. But I think most wouldn't call it classical stats.
Rather than dumping on stats in general, I believe Pearl is just criticizing statistician's reticence to address causal semantics. He considers this a serious problem because of what Carl Sagan calls the "get in and get out" phenomenon, where you drop a study that says "meat consumption 'strongly associated' with increased libido, p < .05" and then bows out knowing full well the two outcomes are going to be causally linked in the mind of the public.
add a comment |
7 Answers
7
active
oldest
votes
7 Answers
7
active
oldest
votes
active
oldest
votes
active
oldest
votes
up vote
41
down vote
accepted
I fully agree that Pearl's tone is arrogant, and his characterisation of "statisticians" is simplistic and monolithic. Also, I don't find his writing particularly clear.
However, I think he has a point.
Causal reasoning was not part of my formal training (MSc): the closest I got to the topic was an elective course in experimental design, i.e. any causality claims required me to physically control the environment. Pearl's book Causality was my first exposure to a refutation of this idea. Obviously I can't speak for all statisticians and curricula, but from my own perspective I subscribe to Pearl's observation that causal reasoning is not a priority in statistics.
It is true that statisticians sometimes control for more variables than is strictly necessary, but this rarely leads to error (at least in my experience).
This is also a belief that I held after graduating with an MSc in statistics in 2010.
However, it is deeply incorrect. When you control for a common effect (called "collider" in the book), you can introduce selection bias. This realization was quite astonishing to me, and really convinced me of the usefulness of representing my causal hypotheses as graphs.
EDIT: I was asked to elaborate on selection bias. This topic is quite subtle, I highly recommend perusing the edX MOOC on Causal Diagrams, a very nice introduction to graphs which has a chapter dedicated to selection bias.
For a toy example, to paraphrase this paper cited in the book: Consider the variables A=attractiveness, B=beauty, C=competence. Suppose that B and C are causally unrelated in the general population (i.e., beauty does not cause competence, competence does not cause beauty, and beauty and competence do not share a common cause). Suppose also that any one of B or C is sufficient for being attractive, i.e. A is a collider. Conditioning on A creates a spurious association between B and C.
A more serious example is the "birth weight paradox", according to which a mother's smoking (S) during pregnancy seems to decrease the mortality (M) of the baby, if the baby is underweight (U). The proposed explanation is that birth defects (D) also cause low birth weight, and also contribute to mortality. The corresponding causal diagram is { S -> U, D -> U, U -> M, S -> M, D -> M } in which U is a collider; conditioning on it introduces the spurious association. The intuition behind this is that if the mother is a smoker, the low birth weight is less likely to be due to a defect.
7
+1. Can you elaborate just a little bit more on how it introduces selection bias? Perhaps a little concrete example will make it clear for most readers.
– amoeba
Nov 15 at 13:57
2
Thanks for the edit. These are very clear examples.
– amoeba
Nov 15 at 23:11
Regarding B and C are unassociated, aren't you misusing the language here? See Section 2.2 on p. 105 in Pearl "Statistics and Causal Inference: A Review" (2003) on language of association vs. causation.
– Richard Hardy
yesterday
1
@RichardHardy : good point -- fixed!
– mitchus
yesterday
add a comment |
up vote
41
down vote
accepted
I fully agree that Pearl's tone is arrogant, and his characterisation of "statisticians" is simplistic and monolithic. Also, I don't find his writing particularly clear.
However, I think he has a point.
Causal reasoning was not part of my formal training (MSc): the closest I got to the topic was an elective course in experimental design, i.e. any causality claims required me to physically control the environment. Pearl's book Causality was my first exposure to a refutation of this idea. Obviously I can't speak for all statisticians and curricula, but from my own perspective I subscribe to Pearl's observation that causal reasoning is not a priority in statistics.
It is true that statisticians sometimes control for more variables than is strictly necessary, but this rarely leads to error (at least in my experience).
This is also a belief that I held after graduating with an MSc in statistics in 2010.
However, it is deeply incorrect. When you control for a common effect (called "collider" in the book), you can introduce selection bias. This realization was quite astonishing to me, and really convinced me of the usefulness of representing my causal hypotheses as graphs.
EDIT: I was asked to elaborate on selection bias. This topic is quite subtle, I highly recommend perusing the edX MOOC on Causal Diagrams, a very nice introduction to graphs which has a chapter dedicated to selection bias.
For a toy example, to paraphrase this paper cited in the book: Consider the variables A=attractiveness, B=beauty, C=competence. Suppose that B and C are causally unrelated in the general population (i.e., beauty does not cause competence, competence does not cause beauty, and beauty and competence do not share a common cause). Suppose also that any one of B or C is sufficient for being attractive, i.e. A is a collider. Conditioning on A creates a spurious association between B and C.
A more serious example is the "birth weight paradox", according to which a mother's smoking (S) during pregnancy seems to decrease the mortality (M) of the baby, if the baby is underweight (U). The proposed explanation is that birth defects (D) also cause low birth weight, and also contribute to mortality. The corresponding causal diagram is { S -> U, D -> U, U -> M, S -> M, D -> M } in which U is a collider; conditioning on it introduces the spurious association. The intuition behind this is that if the mother is a smoker, the low birth weight is less likely to be due to a defect.
7
+1. Can you elaborate just a little bit more on how it introduces selection bias? Perhaps a little concrete example will make it clear for most readers.
– amoeba
Nov 15 at 13:57
2
Thanks for the edit. These are very clear examples.
– amoeba
Nov 15 at 23:11
Regarding B and C are unassociated, aren't you misusing the language here? See Section 2.2 on p. 105 in Pearl "Statistics and Causal Inference: A Review" (2003) on language of association vs. causation.
– Richard Hardy
yesterday
1
@RichardHardy : good point -- fixed!
– mitchus
yesterday
add a comment |
up vote
41
down vote
accepted
up vote
41
down vote
accepted
I fully agree that Pearl's tone is arrogant, and his characterisation of "statisticians" is simplistic and monolithic. Also, I don't find his writing particularly clear.
However, I think he has a point.
Causal reasoning was not part of my formal training (MSc): the closest I got to the topic was an elective course in experimental design, i.e. any causality claims required me to physically control the environment. Pearl's book Causality was my first exposure to a refutation of this idea. Obviously I can't speak for all statisticians and curricula, but from my own perspective I subscribe to Pearl's observation that causal reasoning is not a priority in statistics.
It is true that statisticians sometimes control for more variables than is strictly necessary, but this rarely leads to error (at least in my experience).
This is also a belief that I held after graduating with an MSc in statistics in 2010.
However, it is deeply incorrect. When you control for a common effect (called "collider" in the book), you can introduce selection bias. This realization was quite astonishing to me, and really convinced me of the usefulness of representing my causal hypotheses as graphs.
EDIT: I was asked to elaborate on selection bias. This topic is quite subtle, I highly recommend perusing the edX MOOC on Causal Diagrams, a very nice introduction to graphs which has a chapter dedicated to selection bias.
For a toy example, to paraphrase this paper cited in the book: Consider the variables A=attractiveness, B=beauty, C=competence. Suppose that B and C are causally unrelated in the general population (i.e., beauty does not cause competence, competence does not cause beauty, and beauty and competence do not share a common cause). Suppose also that any one of B or C is sufficient for being attractive, i.e. A is a collider. Conditioning on A creates a spurious association between B and C.
A more serious example is the "birth weight paradox", according to which a mother's smoking (S) during pregnancy seems to decrease the mortality (M) of the baby, if the baby is underweight (U). The proposed explanation is that birth defects (D) also cause low birth weight, and also contribute to mortality. The corresponding causal diagram is { S -> U, D -> U, U -> M, S -> M, D -> M } in which U is a collider; conditioning on it introduces the spurious association. The intuition behind this is that if the mother is a smoker, the low birth weight is less likely to be due to a defect.
I fully agree that Pearl's tone is arrogant, and his characterisation of "statisticians" is simplistic and monolithic. Also, I don't find his writing particularly clear.
However, I think he has a point.
Causal reasoning was not part of my formal training (MSc): the closest I got to the topic was an elective course in experimental design, i.e. any causality claims required me to physically control the environment. Pearl's book Causality was my first exposure to a refutation of this idea. Obviously I can't speak for all statisticians and curricula, but from my own perspective I subscribe to Pearl's observation that causal reasoning is not a priority in statistics.
It is true that statisticians sometimes control for more variables than is strictly necessary, but this rarely leads to error (at least in my experience).
This is also a belief that I held after graduating with an MSc in statistics in 2010.
However, it is deeply incorrect. When you control for a common effect (called "collider" in the book), you can introduce selection bias. This realization was quite astonishing to me, and really convinced me of the usefulness of representing my causal hypotheses as graphs.
EDIT: I was asked to elaborate on selection bias. This topic is quite subtle, I highly recommend perusing the edX MOOC on Causal Diagrams, a very nice introduction to graphs which has a chapter dedicated to selection bias.
For a toy example, to paraphrase this paper cited in the book: Consider the variables A=attractiveness, B=beauty, C=competence. Suppose that B and C are causally unrelated in the general population (i.e., beauty does not cause competence, competence does not cause beauty, and beauty and competence do not share a common cause). Suppose also that any one of B or C is sufficient for being attractive, i.e. A is a collider. Conditioning on A creates a spurious association between B and C.
A more serious example is the "birth weight paradox", according to which a mother's smoking (S) during pregnancy seems to decrease the mortality (M) of the baby, if the baby is underweight (U). The proposed explanation is that birth defects (D) also cause low birth weight, and also contribute to mortality. The corresponding causal diagram is { S -> U, D -> U, U -> M, S -> M, D -> M } in which U is a collider; conditioning on it introduces the spurious association. The intuition behind this is that if the mother is a smoker, the low birth weight is less likely to be due to a defect.
edited yesterday
answered Nov 15 at 13:50
mitchus
55347
55347
7
+1. Can you elaborate just a little bit more on how it introduces selection bias? Perhaps a little concrete example will make it clear for most readers.
– amoeba
Nov 15 at 13:57
2
Thanks for the edit. These are very clear examples.
– amoeba
Nov 15 at 23:11
Regarding B and C are unassociated, aren't you misusing the language here? See Section 2.2 on p. 105 in Pearl "Statistics and Causal Inference: A Review" (2003) on language of association vs. causation.
– Richard Hardy
yesterday
1
@RichardHardy : good point -- fixed!
– mitchus
yesterday
add a comment |
7
+1. Can you elaborate just a little bit more on how it introduces selection bias? Perhaps a little concrete example will make it clear for most readers.
– amoeba
Nov 15 at 13:57
2
Thanks for the edit. These are very clear examples.
– amoeba
Nov 15 at 23:11
Regarding B and C are unassociated, aren't you misusing the language here? See Section 2.2 on p. 105 in Pearl "Statistics and Causal Inference: A Review" (2003) on language of association vs. causation.
– Richard Hardy
yesterday
1
@RichardHardy : good point -- fixed!
– mitchus
yesterday
7
7
+1. Can you elaborate just a little bit more on how it introduces selection bias? Perhaps a little concrete example will make it clear for most readers.
– amoeba
Nov 15 at 13:57
+1. Can you elaborate just a little bit more on how it introduces selection bias? Perhaps a little concrete example will make it clear for most readers.
– amoeba
Nov 15 at 13:57
2
2
Thanks for the edit. These are very clear examples.
– amoeba
Nov 15 at 23:11
Thanks for the edit. These are very clear examples.
– amoeba
Nov 15 at 23:11
Regarding B and C are unassociated, aren't you misusing the language here? See Section 2.2 on p. 105 in Pearl "Statistics and Causal Inference: A Review" (2003) on language of association vs. causation.
– Richard Hardy
yesterday
Regarding B and C are unassociated, aren't you misusing the language here? See Section 2.2 on p. 105 in Pearl "Statistics and Causal Inference: A Review" (2003) on language of association vs. causation.
– Richard Hardy
yesterday
1
1
@RichardHardy : good point -- fixed!
– mitchus
yesterday
@RichardHardy : good point -- fixed!
– mitchus
yesterday
add a comment |
up vote
57
down vote
Your very question reflects what Pearl is saying!
a simple linear regression is essentially a causal model
No, a linear regression is a statistical model, not a causal model. Let's assume $Y, X, Z$ are random variables with a multivariate normal distribution. Then you can correctly estimate the linear expectations $E[Ymid X]$, $E[Xmid Y]$, $E[Ymid X,Z]$, $E[Zmid Y, X]$ etc using linear regression, but there's nothing here that says whether any of those quantities are causal.
A linear structural equation, on the other hand, is a causal model. But the first step is to understand the difference between statistical assumptions (constraints on the observed joint probability distribution) and causal assumptions (constraints on the causal model).
do you think that Judea Pearl misrepresenting statistics, and if yes,
why?
No, I don't think so, because we see these misconceptions daily. Of course, Pearl is making some generalizations, since some statisticians do work with causal inference (Don Rubin was a pioneer in promoting potential outcomes... also, I am a statistician!). But he is correct in saying that the bulk of traditional statistics education shuns causality, even to formally define what a causal effect is.
To make this clear, if we ask a statistician/econometrician with just a regular training to define mathematically what is the expected value of $Y$ if we intervene on $X$, he would probably write $E[Y|X]$ (see an example here)! But that's an observational quantity, that's not how you define a causal effect! In other terms, currently, a student with just a traditional statistics course lacks even the ability of properly defining this quantity mathematically ($E[Y_{x}]$ or $E[Y|do(x)]$) if you are not familiar with the structural/counterfactual theory of causation!
The quote you bring from the book is also a great example. You will not find in traditional statistics books a correct definition of what a confounder is, nor guidance about when you should (or should not) adjust for a covariate in observational studies. In general, you see “correlational criteria”, such as “if the covariate is associated with the treatment and with the outcome, you should adjust for it”. One of the most notable examples of this confusion shows up in Simpson’s Paradox—when faced with two estimates of opposite signs, which one should you use, the adjusted or unadjusted? The, answer, of course, depends on the causal model.
And what does Pearl mean when he says that this question was brought to an end? In the case of simple adjustment via regression, he is referring to the backdoor criterion (see more here). And for identification in general---beyond simple adjustment---he means that we now have complete algorithms for identification of causal effects for any given semi-markovian DAG.
Another remark here is worth making. Even in experimental studies — where traditional statistics has surely done a lot of important work with the design of experiments!— in the end of the day you still need a causal model. Experiments can suffer from lack of compliance, from loss of follow up, from selection bias... also, most of the time you don't want to confine the results of your experiments to the specific population you analyzed, you want to generalize your experimental results to a broader/different population. Here, again, one may ask: what should you adjust for? Are the data and substantive knowledge you have enough to allow such extrapolation? All of these are causal concepts, thus you need a language to formally express causal assumptions and check whether they are enough to allow you to do what you want!
In sum, these misconceptions are widespread in statistics and econometrics, there are several examples here in Cross Validated, such as:
- understanding what a confounder is
- understanding which variables you should include in a regression (for estimating causal effects)
understanding that even if you include all variables a regression may not be causal- understanding the difference between (lack of) statistical associations and causation
- defining a causal model and causal effects
And many more.
Do you think that causal inference is a Revolution with a big R which
really changes all our thinking?
Considering the current state of affairs in many sciences, how much we have advanced and how fast things are changing, and how much we can still do, I would say this is indeed a revolution.
2
Thank you. But – well, writing simplistically, I can calculate E[X|Y] as well as E[Y|X], but I can write X←Y as well as X→Y in a DAG. One way or other, I must start with a scientific hypothesis or a model. My hypothesis, my model – my choice. The very fact that I can do something doesn't mean I should do it, does it.
– January
Nov 14 at 10:28
2
@January it doesn't mean you should, the point here is only about being able to articulate accurately what you want to estimate (the causal estimand), articulate accurately your causal assumptions (making clear the distinction of causal and statistical assumptions), checking the logical implications of those causal assumptions and being able to understand whether your causal assumptions + data are enough for answering your query.
– Carlos Cinelli
Nov 14 at 10:30
3
@January say you have an observational study and want to estimate the causal effect of $X$ on $Y$. How do you decide which covariates to include in your regression?
– Carlos Cinelli
Nov 14 at 10:37
4
I think so: it doesn't seem entirely unfair to suggest that your average statistician, while likely well versed in causal inference from controlled experiments, & certainly in no danger of confusing correlation with causation, might be a bit shaky on causal inference from observational data. I take the last to be the context of the quotation (I haven't read the book) & it's something that some readers of this post may not pick up on.
– Scortchi♦
Nov 14 at 17:15
4
@January In short "adjusting for covariates" does not necessarily mean you have eliminated bias in causal effect estimates from those variables.
– Alexis
Nov 14 at 22:50
|
show 13 more comments
up vote
57
down vote
Your very question reflects what Pearl is saying!
a simple linear regression is essentially a causal model
No, a linear regression is a statistical model, not a causal model. Let's assume $Y, X, Z$ are random variables with a multivariate normal distribution. Then you can correctly estimate the linear expectations $E[Ymid X]$, $E[Xmid Y]$, $E[Ymid X,Z]$, $E[Zmid Y, X]$ etc using linear regression, but there's nothing here that says whether any of those quantities are causal.
A linear structural equation, on the other hand, is a causal model. But the first step is to understand the difference between statistical assumptions (constraints on the observed joint probability distribution) and causal assumptions (constraints on the causal model).
do you think that Judea Pearl misrepresenting statistics, and if yes,
why?
No, I don't think so, because we see these misconceptions daily. Of course, Pearl is making some generalizations, since some statisticians do work with causal inference (Don Rubin was a pioneer in promoting potential outcomes... also, I am a statistician!). But he is correct in saying that the bulk of traditional statistics education shuns causality, even to formally define what a causal effect is.
To make this clear, if we ask a statistician/econometrician with just a regular training to define mathematically what is the expected value of $Y$ if we intervene on $X$, he would probably write $E[Y|X]$ (see an example here)! But that's an observational quantity, that's not how you define a causal effect! In other terms, currently, a student with just a traditional statistics course lacks even the ability of properly defining this quantity mathematically ($E[Y_{x}]$ or $E[Y|do(x)]$) if you are not familiar with the structural/counterfactual theory of causation!
The quote you bring from the book is also a great example. You will not find in traditional statistics books a correct definition of what a confounder is, nor guidance about when you should (or should not) adjust for a covariate in observational studies. In general, you see “correlational criteria”, such as “if the covariate is associated with the treatment and with the outcome, you should adjust for it”. One of the most notable examples of this confusion shows up in Simpson’s Paradox—when faced with two estimates of opposite signs, which one should you use, the adjusted or unadjusted? The, answer, of course, depends on the causal model.
And what does Pearl mean when he says that this question was brought to an end? In the case of simple adjustment via regression, he is referring to the backdoor criterion (see more here). And for identification in general---beyond simple adjustment---he means that we now have complete algorithms for identification of causal effects for any given semi-markovian DAG.
Another remark here is worth making. Even in experimental studies — where traditional statistics has surely done a lot of important work with the design of experiments!— in the end of the day you still need a causal model. Experiments can suffer from lack of compliance, from loss of follow up, from selection bias... also, most of the time you don't want to confine the results of your experiments to the specific population you analyzed, you want to generalize your experimental results to a broader/different population. Here, again, one may ask: what should you adjust for? Are the data and substantive knowledge you have enough to allow such extrapolation? All of these are causal concepts, thus you need a language to formally express causal assumptions and check whether they are enough to allow you to do what you want!
In sum, these misconceptions are widespread in statistics and econometrics, there are several examples here in Cross Validated, such as:
- understanding what a confounder is
- understanding which variables you should include in a regression (for estimating causal effects)
understanding that even if you include all variables a regression may not be causal- understanding the difference between (lack of) statistical associations and causation
- defining a causal model and causal effects
And many more.
Do you think that causal inference is a Revolution with a big R which
really changes all our thinking?
Considering the current state of affairs in many sciences, how much we have advanced and how fast things are changing, and how much we can still do, I would say this is indeed a revolution.
2
Thank you. But – well, writing simplistically, I can calculate E[X|Y] as well as E[Y|X], but I can write X←Y as well as X→Y in a DAG. One way or other, I must start with a scientific hypothesis or a model. My hypothesis, my model – my choice. The very fact that I can do something doesn't mean I should do it, does it.
– January
Nov 14 at 10:28
2
@January it doesn't mean you should, the point here is only about being able to articulate accurately what you want to estimate (the causal estimand), articulate accurately your causal assumptions (making clear the distinction of causal and statistical assumptions), checking the logical implications of those causal assumptions and being able to understand whether your causal assumptions + data are enough for answering your query.
– Carlos Cinelli
Nov 14 at 10:30
3
@January say you have an observational study and want to estimate the causal effect of $X$ on $Y$. How do you decide which covariates to include in your regression?
– Carlos Cinelli
Nov 14 at 10:37
4
I think so: it doesn't seem entirely unfair to suggest that your average statistician, while likely well versed in causal inference from controlled experiments, & certainly in no danger of confusing correlation with causation, might be a bit shaky on causal inference from observational data. I take the last to be the context of the quotation (I haven't read the book) & it's something that some readers of this post may not pick up on.
– Scortchi♦
Nov 14 at 17:15
4
@January In short "adjusting for covariates" does not necessarily mean you have eliminated bias in causal effect estimates from those variables.
– Alexis
Nov 14 at 22:50
|
show 13 more comments
up vote
57
down vote
up vote
57
down vote
Your very question reflects what Pearl is saying!
a simple linear regression is essentially a causal model
No, a linear regression is a statistical model, not a causal model. Let's assume $Y, X, Z$ are random variables with a multivariate normal distribution. Then you can correctly estimate the linear expectations $E[Ymid X]$, $E[Xmid Y]$, $E[Ymid X,Z]$, $E[Zmid Y, X]$ etc using linear regression, but there's nothing here that says whether any of those quantities are causal.
A linear structural equation, on the other hand, is a causal model. But the first step is to understand the difference between statistical assumptions (constraints on the observed joint probability distribution) and causal assumptions (constraints on the causal model).
do you think that Judea Pearl misrepresenting statistics, and if yes,
why?
No, I don't think so, because we see these misconceptions daily. Of course, Pearl is making some generalizations, since some statisticians do work with causal inference (Don Rubin was a pioneer in promoting potential outcomes... also, I am a statistician!). But he is correct in saying that the bulk of traditional statistics education shuns causality, even to formally define what a causal effect is.
To make this clear, if we ask a statistician/econometrician with just a regular training to define mathematically what is the expected value of $Y$ if we intervene on $X$, he would probably write $E[Y|X]$ (see an example here)! But that's an observational quantity, that's not how you define a causal effect! In other terms, currently, a student with just a traditional statistics course lacks even the ability of properly defining this quantity mathematically ($E[Y_{x}]$ or $E[Y|do(x)]$) if you are not familiar with the structural/counterfactual theory of causation!
The quote you bring from the book is also a great example. You will not find in traditional statistics books a correct definition of what a confounder is, nor guidance about when you should (or should not) adjust for a covariate in observational studies. In general, you see “correlational criteria”, such as “if the covariate is associated with the treatment and with the outcome, you should adjust for it”. One of the most notable examples of this confusion shows up in Simpson’s Paradox—when faced with two estimates of opposite signs, which one should you use, the adjusted or unadjusted? The, answer, of course, depends on the causal model.
And what does Pearl mean when he says that this question was brought to an end? In the case of simple adjustment via regression, he is referring to the backdoor criterion (see more here). And for identification in general---beyond simple adjustment---he means that we now have complete algorithms for identification of causal effects for any given semi-markovian DAG.
Another remark here is worth making. Even in experimental studies — where traditional statistics has surely done a lot of important work with the design of experiments!— in the end of the day you still need a causal model. Experiments can suffer from lack of compliance, from loss of follow up, from selection bias... also, most of the time you don't want to confine the results of your experiments to the specific population you analyzed, you want to generalize your experimental results to a broader/different population. Here, again, one may ask: what should you adjust for? Are the data and substantive knowledge you have enough to allow such extrapolation? All of these are causal concepts, thus you need a language to formally express causal assumptions and check whether they are enough to allow you to do what you want!
In sum, these misconceptions are widespread in statistics and econometrics, there are several examples here in Cross Validated, such as:
- understanding what a confounder is
- understanding which variables you should include in a regression (for estimating causal effects)
understanding that even if you include all variables a regression may not be causal- understanding the difference between (lack of) statistical associations and causation
- defining a causal model and causal effects
And many more.
Do you think that causal inference is a Revolution with a big R which
really changes all our thinking?
Considering the current state of affairs in many sciences, how much we have advanced and how fast things are changing, and how much we can still do, I would say this is indeed a revolution.
Your very question reflects what Pearl is saying!
a simple linear regression is essentially a causal model
No, a linear regression is a statistical model, not a causal model. Let's assume $Y, X, Z$ are random variables with a multivariate normal distribution. Then you can correctly estimate the linear expectations $E[Ymid X]$, $E[Xmid Y]$, $E[Ymid X,Z]$, $E[Zmid Y, X]$ etc using linear regression, but there's nothing here that says whether any of those quantities are causal.
A linear structural equation, on the other hand, is a causal model. But the first step is to understand the difference between statistical assumptions (constraints on the observed joint probability distribution) and causal assumptions (constraints on the causal model).
do you think that Judea Pearl misrepresenting statistics, and if yes,
why?
No, I don't think so, because we see these misconceptions daily. Of course, Pearl is making some generalizations, since some statisticians do work with causal inference (Don Rubin was a pioneer in promoting potential outcomes... also, I am a statistician!). But he is correct in saying that the bulk of traditional statistics education shuns causality, even to formally define what a causal effect is.
To make this clear, if we ask a statistician/econometrician with just a regular training to define mathematically what is the expected value of $Y$ if we intervene on $X$, he would probably write $E[Y|X]$ (see an example here)! But that's an observational quantity, that's not how you define a causal effect! In other terms, currently, a student with just a traditional statistics course lacks even the ability of properly defining this quantity mathematically ($E[Y_{x}]$ or $E[Y|do(x)]$) if you are not familiar with the structural/counterfactual theory of causation!
The quote you bring from the book is also a great example. You will not find in traditional statistics books a correct definition of what a confounder is, nor guidance about when you should (or should not) adjust for a covariate in observational studies. In general, you see “correlational criteria”, such as “if the covariate is associated with the treatment and with the outcome, you should adjust for it”. One of the most notable examples of this confusion shows up in Simpson’s Paradox—when faced with two estimates of opposite signs, which one should you use, the adjusted or unadjusted? The, answer, of course, depends on the causal model.
And what does Pearl mean when he says that this question was brought to an end? In the case of simple adjustment via regression, he is referring to the backdoor criterion (see more here). And for identification in general---beyond simple adjustment---he means that we now have complete algorithms for identification of causal effects for any given semi-markovian DAG.
Another remark here is worth making. Even in experimental studies — where traditional statistics has surely done a lot of important work with the design of experiments!— in the end of the day you still need a causal model. Experiments can suffer from lack of compliance, from loss of follow up, from selection bias... also, most of the time you don't want to confine the results of your experiments to the specific population you analyzed, you want to generalize your experimental results to a broader/different population. Here, again, one may ask: what should you adjust for? Are the data and substantive knowledge you have enough to allow such extrapolation? All of these are causal concepts, thus you need a language to formally express causal assumptions and check whether they are enough to allow you to do what you want!
In sum, these misconceptions are widespread in statistics and econometrics, there are several examples here in Cross Validated, such as:
- understanding what a confounder is
- understanding which variables you should include in a regression (for estimating causal effects)
understanding that even if you include all variables a regression may not be causal- understanding the difference between (lack of) statistical associations and causation
- defining a causal model and causal effects
And many more.
Do you think that causal inference is a Revolution with a big R which
really changes all our thinking?
Considering the current state of affairs in many sciences, how much we have advanced and how fast things are changing, and how much we can still do, I would say this is indeed a revolution.
edited Nov 15 at 16:39
Michael Hardy
3,2001330
3,2001330
answered Nov 14 at 9:50


Carlos Cinelli
5,07542146
5,07542146
2
Thank you. But – well, writing simplistically, I can calculate E[X|Y] as well as E[Y|X], but I can write X←Y as well as X→Y in a DAG. One way or other, I must start with a scientific hypothesis or a model. My hypothesis, my model – my choice. The very fact that I can do something doesn't mean I should do it, does it.
– January
Nov 14 at 10:28
2
@January it doesn't mean you should, the point here is only about being able to articulate accurately what you want to estimate (the causal estimand), articulate accurately your causal assumptions (making clear the distinction of causal and statistical assumptions), checking the logical implications of those causal assumptions and being able to understand whether your causal assumptions + data are enough for answering your query.
– Carlos Cinelli
Nov 14 at 10:30
3
@January say you have an observational study and want to estimate the causal effect of $X$ on $Y$. How do you decide which covariates to include in your regression?
– Carlos Cinelli
Nov 14 at 10:37
4
I think so: it doesn't seem entirely unfair to suggest that your average statistician, while likely well versed in causal inference from controlled experiments, & certainly in no danger of confusing correlation with causation, might be a bit shaky on causal inference from observational data. I take the last to be the context of the quotation (I haven't read the book) & it's something that some readers of this post may not pick up on.
– Scortchi♦
Nov 14 at 17:15
4
@January In short "adjusting for covariates" does not necessarily mean you have eliminated bias in causal effect estimates from those variables.
– Alexis
Nov 14 at 22:50
|
show 13 more comments
2
Thank you. But – well, writing simplistically, I can calculate E[X|Y] as well as E[Y|X], but I can write X←Y as well as X→Y in a DAG. One way or other, I must start with a scientific hypothesis or a model. My hypothesis, my model – my choice. The very fact that I can do something doesn't mean I should do it, does it.
– January
Nov 14 at 10:28
2
@January it doesn't mean you should, the point here is only about being able to articulate accurately what you want to estimate (the causal estimand), articulate accurately your causal assumptions (making clear the distinction of causal and statistical assumptions), checking the logical implications of those causal assumptions and being able to understand whether your causal assumptions + data are enough for answering your query.
– Carlos Cinelli
Nov 14 at 10:30
3
@January say you have an observational study and want to estimate the causal effect of $X$ on $Y$. How do you decide which covariates to include in your regression?
– Carlos Cinelli
Nov 14 at 10:37
4
I think so: it doesn't seem entirely unfair to suggest that your average statistician, while likely well versed in causal inference from controlled experiments, & certainly in no danger of confusing correlation with causation, might be a bit shaky on causal inference from observational data. I take the last to be the context of the quotation (I haven't read the book) & it's something that some readers of this post may not pick up on.
– Scortchi♦
Nov 14 at 17:15
4
@January In short "adjusting for covariates" does not necessarily mean you have eliminated bias in causal effect estimates from those variables.
– Alexis
Nov 14 at 22:50
2
2
Thank you. But – well, writing simplistically, I can calculate E[X|Y] as well as E[Y|X], but I can write X←Y as well as X→Y in a DAG. One way or other, I must start with a scientific hypothesis or a model. My hypothesis, my model – my choice. The very fact that I can do something doesn't mean I should do it, does it.
– January
Nov 14 at 10:28
Thank you. But – well, writing simplistically, I can calculate E[X|Y] as well as E[Y|X], but I can write X←Y as well as X→Y in a DAG. One way or other, I must start with a scientific hypothesis or a model. My hypothesis, my model – my choice. The very fact that I can do something doesn't mean I should do it, does it.
– January
Nov 14 at 10:28
2
2
@January it doesn't mean you should, the point here is only about being able to articulate accurately what you want to estimate (the causal estimand), articulate accurately your causal assumptions (making clear the distinction of causal and statistical assumptions), checking the logical implications of those causal assumptions and being able to understand whether your causal assumptions + data are enough for answering your query.
– Carlos Cinelli
Nov 14 at 10:30
@January it doesn't mean you should, the point here is only about being able to articulate accurately what you want to estimate (the causal estimand), articulate accurately your causal assumptions (making clear the distinction of causal and statistical assumptions), checking the logical implications of those causal assumptions and being able to understand whether your causal assumptions + data are enough for answering your query.
– Carlos Cinelli
Nov 14 at 10:30
3
3
@January say you have an observational study and want to estimate the causal effect of $X$ on $Y$. How do you decide which covariates to include in your regression?
– Carlos Cinelli
Nov 14 at 10:37
@January say you have an observational study and want to estimate the causal effect of $X$ on $Y$. How do you decide which covariates to include in your regression?
– Carlos Cinelli
Nov 14 at 10:37
4
4
I think so: it doesn't seem entirely unfair to suggest that your average statistician, while likely well versed in causal inference from controlled experiments, & certainly in no danger of confusing correlation with causation, might be a bit shaky on causal inference from observational data. I take the last to be the context of the quotation (I haven't read the book) & it's something that some readers of this post may not pick up on.
– Scortchi♦
Nov 14 at 17:15
I think so: it doesn't seem entirely unfair to suggest that your average statistician, while likely well versed in causal inference from controlled experiments, & certainly in no danger of confusing correlation with causation, might be a bit shaky on causal inference from observational data. I take the last to be the context of the quotation (I haven't read the book) & it's something that some readers of this post may not pick up on.
– Scortchi♦
Nov 14 at 17:15
4
4
@January In short "adjusting for covariates" does not necessarily mean you have eliminated bias in causal effect estimates from those variables.
– Alexis
Nov 14 at 22:50
@January In short "adjusting for covariates" does not necessarily mean you have eliminated bias in causal effect estimates from those variables.
– Alexis
Nov 14 at 22:50
|
show 13 more comments
up vote
24
down vote
I'm a fan of Judea's writing, and I've read Causality (love) and Book of Why (like).
I do not feel that Judea is bashing statistics. It's hard to hear criticism. But what can we say about any person or field that doesn't take criticism? They tend from greatness to complacency. You must ask: is the criticism correct, needed, useful, and does it propose alternatives? The answer to all those is an emphatic "Yes".
Correct? I've reviewed and collaborated on a few dozen papers, mostly analyses of observational data, and I rarely feel there is a sufficient discussion of causality. The "adjustment" approach involves selecting variables because they were hand-picked from the DD as being "useful" "relevant" "important" or other nonsense.$^1$
Needed? The media is awash with seemingly contradictory statements about the health effects of major exposures. Inconsistency with data analysis has stagnated evidence which leaves us lacking useful policy, healthcare procedures, and recommendations for better living.
Useful? Judea's comment is pertinent and specific enough to give pause. It is directly relevant to any data analysis any statistician or data expert might encounter.
Does it propose alternatives? Yes, Judea in fact discusses the possibility of advanced statistical methods, and even how they reduce to known statistical frameworks (like Structural Equation Modeling) and their connection to regression models). It all boils down to requiring an explicit statement of the content knowledge that has guided the modeling approach.
Judea isn't simply suggesting we defenestrate all statistical methods (e.g. regression). Rather, he is saying that we need to embrace some causal theory to justify models.
$^1$ the complaint here is about the use of convincing and imprecise language to justify what is ultimately the wrong approach to modeling. There can be overlap, serendipitously, but Pearl is clear about the purpose of a causal diagram (DAG) and how variables can be classified as "confounders".
3
Nice answer. Note that not being a statistician but having served as an interface between statistics and biology for many years, for me any criticism of statisticians is really not so hard to hear ;-) However, do you really think that "orthodox statistics" cannot handle causality at all, as Pearl explicitly states?
– January
Nov 14 at 15:11
3
@January au contraire. I think that the deficiency among statisticians in accepting the causal inference in their analyses is directly related to their deficiency in understanding frequentist inference. It's the counterfactual reasoning that lacks.
– AdamO
Nov 14 at 15:38
4
+1 "The "adjustment" approach involves selecting variables because they were hand-picked from the DD as being "useful" "relevant" "important" or other nonsense without actually incorporating formal hypotheses about the specific causal relationships among them (a la the formal use of DAGs) ." Edit added. :)
– Alexis
Nov 14 at 21:15
Comments are not for extended discussion; this conversation has been moved to chat.
– Scortchi♦
Nov 15 at 23:17
add a comment |
up vote
24
down vote
I'm a fan of Judea's writing, and I've read Causality (love) and Book of Why (like).
I do not feel that Judea is bashing statistics. It's hard to hear criticism. But what can we say about any person or field that doesn't take criticism? They tend from greatness to complacency. You must ask: is the criticism correct, needed, useful, and does it propose alternatives? The answer to all those is an emphatic "Yes".
Correct? I've reviewed and collaborated on a few dozen papers, mostly analyses of observational data, and I rarely feel there is a sufficient discussion of causality. The "adjustment" approach involves selecting variables because they were hand-picked from the DD as being "useful" "relevant" "important" or other nonsense.$^1$
Needed? The media is awash with seemingly contradictory statements about the health effects of major exposures. Inconsistency with data analysis has stagnated evidence which leaves us lacking useful policy, healthcare procedures, and recommendations for better living.
Useful? Judea's comment is pertinent and specific enough to give pause. It is directly relevant to any data analysis any statistician or data expert might encounter.
Does it propose alternatives? Yes, Judea in fact discusses the possibility of advanced statistical methods, and even how they reduce to known statistical frameworks (like Structural Equation Modeling) and their connection to regression models). It all boils down to requiring an explicit statement of the content knowledge that has guided the modeling approach.
Judea isn't simply suggesting we defenestrate all statistical methods (e.g. regression). Rather, he is saying that we need to embrace some causal theory to justify models.
$^1$ the complaint here is about the use of convincing and imprecise language to justify what is ultimately the wrong approach to modeling. There can be overlap, serendipitously, but Pearl is clear about the purpose of a causal diagram (DAG) and how variables can be classified as "confounders".
3
Nice answer. Note that not being a statistician but having served as an interface between statistics and biology for many years, for me any criticism of statisticians is really not so hard to hear ;-) However, do you really think that "orthodox statistics" cannot handle causality at all, as Pearl explicitly states?
– January
Nov 14 at 15:11
3
@January au contraire. I think that the deficiency among statisticians in accepting the causal inference in their analyses is directly related to their deficiency in understanding frequentist inference. It's the counterfactual reasoning that lacks.
– AdamO
Nov 14 at 15:38
4
+1 "The "adjustment" approach involves selecting variables because they were hand-picked from the DD as being "useful" "relevant" "important" or other nonsense without actually incorporating formal hypotheses about the specific causal relationships among them (a la the formal use of DAGs) ." Edit added. :)
– Alexis
Nov 14 at 21:15
Comments are not for extended discussion; this conversation has been moved to chat.
– Scortchi♦
Nov 15 at 23:17
add a comment |
up vote
24
down vote
up vote
24
down vote
I'm a fan of Judea's writing, and I've read Causality (love) and Book of Why (like).
I do not feel that Judea is bashing statistics. It's hard to hear criticism. But what can we say about any person or field that doesn't take criticism? They tend from greatness to complacency. You must ask: is the criticism correct, needed, useful, and does it propose alternatives? The answer to all those is an emphatic "Yes".
Correct? I've reviewed and collaborated on a few dozen papers, mostly analyses of observational data, and I rarely feel there is a sufficient discussion of causality. The "adjustment" approach involves selecting variables because they were hand-picked from the DD as being "useful" "relevant" "important" or other nonsense.$^1$
Needed? The media is awash with seemingly contradictory statements about the health effects of major exposures. Inconsistency with data analysis has stagnated evidence which leaves us lacking useful policy, healthcare procedures, and recommendations for better living.
Useful? Judea's comment is pertinent and specific enough to give pause. It is directly relevant to any data analysis any statistician or data expert might encounter.
Does it propose alternatives? Yes, Judea in fact discusses the possibility of advanced statistical methods, and even how they reduce to known statistical frameworks (like Structural Equation Modeling) and their connection to regression models). It all boils down to requiring an explicit statement of the content knowledge that has guided the modeling approach.
Judea isn't simply suggesting we defenestrate all statistical methods (e.g. regression). Rather, he is saying that we need to embrace some causal theory to justify models.
$^1$ the complaint here is about the use of convincing and imprecise language to justify what is ultimately the wrong approach to modeling. There can be overlap, serendipitously, but Pearl is clear about the purpose of a causal diagram (DAG) and how variables can be classified as "confounders".
I'm a fan of Judea's writing, and I've read Causality (love) and Book of Why (like).
I do not feel that Judea is bashing statistics. It's hard to hear criticism. But what can we say about any person or field that doesn't take criticism? They tend from greatness to complacency. You must ask: is the criticism correct, needed, useful, and does it propose alternatives? The answer to all those is an emphatic "Yes".
Correct? I've reviewed and collaborated on a few dozen papers, mostly analyses of observational data, and I rarely feel there is a sufficient discussion of causality. The "adjustment" approach involves selecting variables because they were hand-picked from the DD as being "useful" "relevant" "important" or other nonsense.$^1$
Needed? The media is awash with seemingly contradictory statements about the health effects of major exposures. Inconsistency with data analysis has stagnated evidence which leaves us lacking useful policy, healthcare procedures, and recommendations for better living.
Useful? Judea's comment is pertinent and specific enough to give pause. It is directly relevant to any data analysis any statistician or data expert might encounter.
Does it propose alternatives? Yes, Judea in fact discusses the possibility of advanced statistical methods, and even how they reduce to known statistical frameworks (like Structural Equation Modeling) and their connection to regression models). It all boils down to requiring an explicit statement of the content knowledge that has guided the modeling approach.
Judea isn't simply suggesting we defenestrate all statistical methods (e.g. regression). Rather, he is saying that we need to embrace some causal theory to justify models.
$^1$ the complaint here is about the use of convincing and imprecise language to justify what is ultimately the wrong approach to modeling. There can be overlap, serendipitously, but Pearl is clear about the purpose of a causal diagram (DAG) and how variables can be classified as "confounders".
edited Nov 14 at 21:23
answered Nov 14 at 15:00
AdamO
31.6k256134
31.6k256134
3
Nice answer. Note that not being a statistician but having served as an interface between statistics and biology for many years, for me any criticism of statisticians is really not so hard to hear ;-) However, do you really think that "orthodox statistics" cannot handle causality at all, as Pearl explicitly states?
– January
Nov 14 at 15:11
3
@January au contraire. I think that the deficiency among statisticians in accepting the causal inference in their analyses is directly related to their deficiency in understanding frequentist inference. It's the counterfactual reasoning that lacks.
– AdamO
Nov 14 at 15:38
4
+1 "The "adjustment" approach involves selecting variables because they were hand-picked from the DD as being "useful" "relevant" "important" or other nonsense without actually incorporating formal hypotheses about the specific causal relationships among them (a la the formal use of DAGs) ." Edit added. :)
– Alexis
Nov 14 at 21:15
Comments are not for extended discussion; this conversation has been moved to chat.
– Scortchi♦
Nov 15 at 23:17
add a comment |
3
Nice answer. Note that not being a statistician but having served as an interface between statistics and biology for many years, for me any criticism of statisticians is really not so hard to hear ;-) However, do you really think that "orthodox statistics" cannot handle causality at all, as Pearl explicitly states?
– January
Nov 14 at 15:11
3
@January au contraire. I think that the deficiency among statisticians in accepting the causal inference in their analyses is directly related to their deficiency in understanding frequentist inference. It's the counterfactual reasoning that lacks.
– AdamO
Nov 14 at 15:38
4
+1 "The "adjustment" approach involves selecting variables because they were hand-picked from the DD as being "useful" "relevant" "important" or other nonsense without actually incorporating formal hypotheses about the specific causal relationships among them (a la the formal use of DAGs) ." Edit added. :)
– Alexis
Nov 14 at 21:15
Comments are not for extended discussion; this conversation has been moved to chat.
– Scortchi♦
Nov 15 at 23:17
3
3
Nice answer. Note that not being a statistician but having served as an interface between statistics and biology for many years, for me any criticism of statisticians is really not so hard to hear ;-) However, do you really think that "orthodox statistics" cannot handle causality at all, as Pearl explicitly states?
– January
Nov 14 at 15:11
Nice answer. Note that not being a statistician but having served as an interface between statistics and biology for many years, for me any criticism of statisticians is really not so hard to hear ;-) However, do you really think that "orthodox statistics" cannot handle causality at all, as Pearl explicitly states?
– January
Nov 14 at 15:11
3
3
@January au contraire. I think that the deficiency among statisticians in accepting the causal inference in their analyses is directly related to their deficiency in understanding frequentist inference. It's the counterfactual reasoning that lacks.
– AdamO
Nov 14 at 15:38
@January au contraire. I think that the deficiency among statisticians in accepting the causal inference in their analyses is directly related to their deficiency in understanding frequentist inference. It's the counterfactual reasoning that lacks.
– AdamO
Nov 14 at 15:38
4
4
+1 "The "adjustment" approach involves selecting variables because they were hand-picked from the DD as being "useful" "relevant" "important" or other nonsense without actually incorporating formal hypotheses about the specific causal relationships among them (a la the formal use of DAGs) ." Edit added. :)
– Alexis
Nov 14 at 21:15
+1 "The "adjustment" approach involves selecting variables because they were hand-picked from the DD as being "useful" "relevant" "important" or other nonsense without actually incorporating formal hypotheses about the specific causal relationships among them (a la the formal use of DAGs) ." Edit added. :)
– Alexis
Nov 14 at 21:15
Comments are not for extended discussion; this conversation has been moved to chat.
– Scortchi♦
Nov 15 at 23:17
Comments are not for extended discussion; this conversation has been moved to chat.
– Scortchi♦
Nov 15 at 23:17
add a comment |
up vote
19
down vote
I haven't read this book, so I can only judge the particular quote you give. However, even on this basis, I agree with you that this seems extremely unfair to the statistical profession. I actually think that statisticians have always done a remarkably good job at stressing the distinction between statistical associations (correlation, etc.) and causality, and warning against the conflation of the two. Indeed, in my experience, statisticians have generally been the primary professional force fighting against the ubiquitous confusion between cause and correlation. It is outright false (and virtually slander) to claim that statisticians are "...loath to talk about causality at all." I can see why you are annoyed reading arrogant horseshit like this.
I would say that it is reasonably common for non-statisticians who use statistical models to have a poor understanding of the relationship between statistical association and causality. Some have good scientific training from other fields, in which case they may also be well aware of the issue, but there are certainly some people who use statistical models who have a poor grasp of these issues. This is true in many applied scientific fields where practitioners have basic training in statistics, but do not learn it at a deep level. In these cases it is often professional statisticians who alert other researchers to the distinctions between these concepts and their proper relationship. Statisticians are often the key designers of RCTs and other experiments involving controls used to isolate causality. They are often called on to explain protocols such as randomisation, placebos, and other protocols that are used to try to sever relationships with potential confounding variables. It is true that statisticians sometimes control for more variables than is strictly necessary, but this rarely leads to error (at least in my experience).
I think Judea Pearl has made a very valuable contribution to statistics with his work on causality, and I am grateful to him for this wonderful contribution. He has constructed and examined some very useful formalisms that help to isolate causal relationships, and his work has become a staple of a good statistical education. I read his book Causality while I was a grad student, and it is on my shelf, and on the shelves of many other statisticians. Much of this formalism echoes things that have been known intuitively to statisticians since before they were formalised into an algebraic system, but it is very valuable in any case, and goes beyond that which is obvious. (I actually think in the future we will see a merging of the "do" operation with probability algebra occurring at an axiomatic level, and this will probably eventually become the core of probability theory. I would love to see this built directly into statistical education, so that you learn about causal models and the "do" operation when you learn about probability measures.)
One final thing to bear in mind here is that there are many applications of statistics where the goal is predictive, where the practitioner is not seeking to infer causality. These types of applications are extremely common in statistics, and in such cases, it is important not to restrict oneself to causal relationships. This is true in most applications of statistics in finance, HR, workforce modelling, and many other fields. One should not underestimate the amount of contexts where one cannot or should not seek to control variables.
Update: I notice that my answer disagrees with the one provided by Carlos. Perhaps we disagree on what constitutes "a statistician/econometrician with just a regular training". Anyone who I would call a "statistician" usually has at least a graduate-level education, and usually has substantial professional training/experience. (For example, in Australia, the requirement to become an "Accredited Statistician" with our national professional body requires a minimum of four years experience after an honours degree, or six years experience after a regular bachelors degree.) In any case, a student studying statistics is not a statistician.
I notice that as evidence of the alleged lack of understanding of causality by statisticians, Carlos's answer points to several questions on CV.SE which ask about causality in regression. In every one of these cases, the question is asked by someone who is obviously a novice (not a statistician) and the answers given by Carlos and others (which reflect the correct explanation) are highly-upvoted answers. Indeed, in several of the cases Carlos has given a detailed account of the causality and his answers are the most highly up-voted. This surely proves that statisticians do understand causality.
12
I am a non-statistician whose formal training in statistics was by non-statisticians in the same field, and I teach and research with non-statisticians applying statistics. I can assure you that the principle that (e.g.) correlation is not causation is, and was, a recurrent mantra in my field. Indeed I don't come across people who can't see that a correlation between rainfall and wheat yield isn't all that needs to be said about the relationship between them and the underlying processes. Typically, in my experience, non-statisticians too have thought this through long since.
– Nick Cox
Nov 14 at 12:49
7
As an epidemiologist, I'm getting more and more annoyed by this mantra. As @NickCox says, this is something that even non-scientists understand. The problem I have is when everyone jumps on the bandwagon of "correlation does not mean causation!" whenever an observational study (a case-control study, say) is published. Yes, correlation does not mean causation but the researchers are usually quite aware of that and will do everything to design and analyze a study in such a way that a causal interpretation is at least plausible.
– COOLSerdash
Nov 14 at 12:59
4
@Nick Cox: I have edited to more accurately state that there are many non-statisticians who understand this well. It was not my intention to cast dispersions over other professions - only to stress that the issue is extremely well understood by statisticians.
– Ben
Nov 14 at 13:00
7
@NickCox There's a lot more to Pearl's contributions about causality than "correlation is not causation". I'm with Carlos here. There's enough to learn about causality that it should be a whole course. As far as I know, most statistics departments don't offer such a course.
– Neil G
Nov 15 at 0:35
11
@Ben : Pearl does not accuse statisticians of confusing correlation and causation. He accuses them of mostly steering clear of causal reasoning. I agree with you that his tone is arrogant, but I think he has a point.
– mitchus
Nov 15 at 13:19
|
show 9 more comments
up vote
19
down vote
I haven't read this book, so I can only judge the particular quote you give. However, even on this basis, I agree with you that this seems extremely unfair to the statistical profession. I actually think that statisticians have always done a remarkably good job at stressing the distinction between statistical associations (correlation, etc.) and causality, and warning against the conflation of the two. Indeed, in my experience, statisticians have generally been the primary professional force fighting against the ubiquitous confusion between cause and correlation. It is outright false (and virtually slander) to claim that statisticians are "...loath to talk about causality at all." I can see why you are annoyed reading arrogant horseshit like this.
I would say that it is reasonably common for non-statisticians who use statistical models to have a poor understanding of the relationship between statistical association and causality. Some have good scientific training from other fields, in which case they may also be well aware of the issue, but there are certainly some people who use statistical models who have a poor grasp of these issues. This is true in many applied scientific fields where practitioners have basic training in statistics, but do not learn it at a deep level. In these cases it is often professional statisticians who alert other researchers to the distinctions between these concepts and their proper relationship. Statisticians are often the key designers of RCTs and other experiments involving controls used to isolate causality. They are often called on to explain protocols such as randomisation, placebos, and other protocols that are used to try to sever relationships with potential confounding variables. It is true that statisticians sometimes control for more variables than is strictly necessary, but this rarely leads to error (at least in my experience).
I think Judea Pearl has made a very valuable contribution to statistics with his work on causality, and I am grateful to him for this wonderful contribution. He has constructed and examined some very useful formalisms that help to isolate causal relationships, and his work has become a staple of a good statistical education. I read his book Causality while I was a grad student, and it is on my shelf, and on the shelves of many other statisticians. Much of this formalism echoes things that have been known intuitively to statisticians since before they were formalised into an algebraic system, but it is very valuable in any case, and goes beyond that which is obvious. (I actually think in the future we will see a merging of the "do" operation with probability algebra occurring at an axiomatic level, and this will probably eventually become the core of probability theory. I would love to see this built directly into statistical education, so that you learn about causal models and the "do" operation when you learn about probability measures.)
One final thing to bear in mind here is that there are many applications of statistics where the goal is predictive, where the practitioner is not seeking to infer causality. These types of applications are extremely common in statistics, and in such cases, it is important not to restrict oneself to causal relationships. This is true in most applications of statistics in finance, HR, workforce modelling, and many other fields. One should not underestimate the amount of contexts where one cannot or should not seek to control variables.
Update: I notice that my answer disagrees with the one provided by Carlos. Perhaps we disagree on what constitutes "a statistician/econometrician with just a regular training". Anyone who I would call a "statistician" usually has at least a graduate-level education, and usually has substantial professional training/experience. (For example, in Australia, the requirement to become an "Accredited Statistician" with our national professional body requires a minimum of four years experience after an honours degree, or six years experience after a regular bachelors degree.) In any case, a student studying statistics is not a statistician.
I notice that as evidence of the alleged lack of understanding of causality by statisticians, Carlos's answer points to several questions on CV.SE which ask about causality in regression. In every one of these cases, the question is asked by someone who is obviously a novice (not a statistician) and the answers given by Carlos and others (which reflect the correct explanation) are highly-upvoted answers. Indeed, in several of the cases Carlos has given a detailed account of the causality and his answers are the most highly up-voted. This surely proves that statisticians do understand causality.
12
I am a non-statistician whose formal training in statistics was by non-statisticians in the same field, and I teach and research with non-statisticians applying statistics. I can assure you that the principle that (e.g.) correlation is not causation is, and was, a recurrent mantra in my field. Indeed I don't come across people who can't see that a correlation between rainfall and wheat yield isn't all that needs to be said about the relationship between them and the underlying processes. Typically, in my experience, non-statisticians too have thought this through long since.
– Nick Cox
Nov 14 at 12:49
7
As an epidemiologist, I'm getting more and more annoyed by this mantra. As @NickCox says, this is something that even non-scientists understand. The problem I have is when everyone jumps on the bandwagon of "correlation does not mean causation!" whenever an observational study (a case-control study, say) is published. Yes, correlation does not mean causation but the researchers are usually quite aware of that and will do everything to design and analyze a study in such a way that a causal interpretation is at least plausible.
– COOLSerdash
Nov 14 at 12:59
4
@Nick Cox: I have edited to more accurately state that there are many non-statisticians who understand this well. It was not my intention to cast dispersions over other professions - only to stress that the issue is extremely well understood by statisticians.
– Ben
Nov 14 at 13:00
7
@NickCox There's a lot more to Pearl's contributions about causality than "correlation is not causation". I'm with Carlos here. There's enough to learn about causality that it should be a whole course. As far as I know, most statistics departments don't offer such a course.
– Neil G
Nov 15 at 0:35
11
@Ben : Pearl does not accuse statisticians of confusing correlation and causation. He accuses them of mostly steering clear of causal reasoning. I agree with you that his tone is arrogant, but I think he has a point.
– mitchus
Nov 15 at 13:19
|
show 9 more comments
up vote
19
down vote
up vote
19
down vote
I haven't read this book, so I can only judge the particular quote you give. However, even on this basis, I agree with you that this seems extremely unfair to the statistical profession. I actually think that statisticians have always done a remarkably good job at stressing the distinction between statistical associations (correlation, etc.) and causality, and warning against the conflation of the two. Indeed, in my experience, statisticians have generally been the primary professional force fighting against the ubiquitous confusion between cause and correlation. It is outright false (and virtually slander) to claim that statisticians are "...loath to talk about causality at all." I can see why you are annoyed reading arrogant horseshit like this.
I would say that it is reasonably common for non-statisticians who use statistical models to have a poor understanding of the relationship between statistical association and causality. Some have good scientific training from other fields, in which case they may also be well aware of the issue, but there are certainly some people who use statistical models who have a poor grasp of these issues. This is true in many applied scientific fields where practitioners have basic training in statistics, but do not learn it at a deep level. In these cases it is often professional statisticians who alert other researchers to the distinctions between these concepts and their proper relationship. Statisticians are often the key designers of RCTs and other experiments involving controls used to isolate causality. They are often called on to explain protocols such as randomisation, placebos, and other protocols that are used to try to sever relationships with potential confounding variables. It is true that statisticians sometimes control for more variables than is strictly necessary, but this rarely leads to error (at least in my experience).
I think Judea Pearl has made a very valuable contribution to statistics with his work on causality, and I am grateful to him for this wonderful contribution. He has constructed and examined some very useful formalisms that help to isolate causal relationships, and his work has become a staple of a good statistical education. I read his book Causality while I was a grad student, and it is on my shelf, and on the shelves of many other statisticians. Much of this formalism echoes things that have been known intuitively to statisticians since before they were formalised into an algebraic system, but it is very valuable in any case, and goes beyond that which is obvious. (I actually think in the future we will see a merging of the "do" operation with probability algebra occurring at an axiomatic level, and this will probably eventually become the core of probability theory. I would love to see this built directly into statistical education, so that you learn about causal models and the "do" operation when you learn about probability measures.)
One final thing to bear in mind here is that there are many applications of statistics where the goal is predictive, where the practitioner is not seeking to infer causality. These types of applications are extremely common in statistics, and in such cases, it is important not to restrict oneself to causal relationships. This is true in most applications of statistics in finance, HR, workforce modelling, and many other fields. One should not underestimate the amount of contexts where one cannot or should not seek to control variables.
Update: I notice that my answer disagrees with the one provided by Carlos. Perhaps we disagree on what constitutes "a statistician/econometrician with just a regular training". Anyone who I would call a "statistician" usually has at least a graduate-level education, and usually has substantial professional training/experience. (For example, in Australia, the requirement to become an "Accredited Statistician" with our national professional body requires a minimum of four years experience after an honours degree, or six years experience after a regular bachelors degree.) In any case, a student studying statistics is not a statistician.
I notice that as evidence of the alleged lack of understanding of causality by statisticians, Carlos's answer points to several questions on CV.SE which ask about causality in regression. In every one of these cases, the question is asked by someone who is obviously a novice (not a statistician) and the answers given by Carlos and others (which reflect the correct explanation) are highly-upvoted answers. Indeed, in several of the cases Carlos has given a detailed account of the causality and his answers are the most highly up-voted. This surely proves that statisticians do understand causality.
I haven't read this book, so I can only judge the particular quote you give. However, even on this basis, I agree with you that this seems extremely unfair to the statistical profession. I actually think that statisticians have always done a remarkably good job at stressing the distinction between statistical associations (correlation, etc.) and causality, and warning against the conflation of the two. Indeed, in my experience, statisticians have generally been the primary professional force fighting against the ubiquitous confusion between cause and correlation. It is outright false (and virtually slander) to claim that statisticians are "...loath to talk about causality at all." I can see why you are annoyed reading arrogant horseshit like this.
I would say that it is reasonably common for non-statisticians who use statistical models to have a poor understanding of the relationship between statistical association and causality. Some have good scientific training from other fields, in which case they may also be well aware of the issue, but there are certainly some people who use statistical models who have a poor grasp of these issues. This is true in many applied scientific fields where practitioners have basic training in statistics, but do not learn it at a deep level. In these cases it is often professional statisticians who alert other researchers to the distinctions between these concepts and their proper relationship. Statisticians are often the key designers of RCTs and other experiments involving controls used to isolate causality. They are often called on to explain protocols such as randomisation, placebos, and other protocols that are used to try to sever relationships with potential confounding variables. It is true that statisticians sometimes control for more variables than is strictly necessary, but this rarely leads to error (at least in my experience).
I think Judea Pearl has made a very valuable contribution to statistics with his work on causality, and I am grateful to him for this wonderful contribution. He has constructed and examined some very useful formalisms that help to isolate causal relationships, and his work has become a staple of a good statistical education. I read his book Causality while I was a grad student, and it is on my shelf, and on the shelves of many other statisticians. Much of this formalism echoes things that have been known intuitively to statisticians since before they were formalised into an algebraic system, but it is very valuable in any case, and goes beyond that which is obvious. (I actually think in the future we will see a merging of the "do" operation with probability algebra occurring at an axiomatic level, and this will probably eventually become the core of probability theory. I would love to see this built directly into statistical education, so that you learn about causal models and the "do" operation when you learn about probability measures.)
One final thing to bear in mind here is that there are many applications of statistics where the goal is predictive, where the practitioner is not seeking to infer causality. These types of applications are extremely common in statistics, and in such cases, it is important not to restrict oneself to causal relationships. This is true in most applications of statistics in finance, HR, workforce modelling, and many other fields. One should not underestimate the amount of contexts where one cannot or should not seek to control variables.
Update: I notice that my answer disagrees with the one provided by Carlos. Perhaps we disagree on what constitutes "a statistician/econometrician with just a regular training". Anyone who I would call a "statistician" usually has at least a graduate-level education, and usually has substantial professional training/experience. (For example, in Australia, the requirement to become an "Accredited Statistician" with our national professional body requires a minimum of four years experience after an honours degree, or six years experience after a regular bachelors degree.) In any case, a student studying statistics is not a statistician.
I notice that as evidence of the alleged lack of understanding of causality by statisticians, Carlos's answer points to several questions on CV.SE which ask about causality in regression. In every one of these cases, the question is asked by someone who is obviously a novice (not a statistician) and the answers given by Carlos and others (which reflect the correct explanation) are highly-upvoted answers. Indeed, in several of the cases Carlos has given a detailed account of the causality and his answers are the most highly up-voted. This surely proves that statisticians do understand causality.
edited Nov 14 at 12:58
answered Nov 14 at 12:34


Ben
18.4k22290
18.4k22290
12
I am a non-statistician whose formal training in statistics was by non-statisticians in the same field, and I teach and research with non-statisticians applying statistics. I can assure you that the principle that (e.g.) correlation is not causation is, and was, a recurrent mantra in my field. Indeed I don't come across people who can't see that a correlation between rainfall and wheat yield isn't all that needs to be said about the relationship between them and the underlying processes. Typically, in my experience, non-statisticians too have thought this through long since.
– Nick Cox
Nov 14 at 12:49
7
As an epidemiologist, I'm getting more and more annoyed by this mantra. As @NickCox says, this is something that even non-scientists understand. The problem I have is when everyone jumps on the bandwagon of "correlation does not mean causation!" whenever an observational study (a case-control study, say) is published. Yes, correlation does not mean causation but the researchers are usually quite aware of that and will do everything to design and analyze a study in such a way that a causal interpretation is at least plausible.
– COOLSerdash
Nov 14 at 12:59
4
@Nick Cox: I have edited to more accurately state that there are many non-statisticians who understand this well. It was not my intention to cast dispersions over other professions - only to stress that the issue is extremely well understood by statisticians.
– Ben
Nov 14 at 13:00
7
@NickCox There's a lot more to Pearl's contributions about causality than "correlation is not causation". I'm with Carlos here. There's enough to learn about causality that it should be a whole course. As far as I know, most statistics departments don't offer such a course.
– Neil G
Nov 15 at 0:35
11
@Ben : Pearl does not accuse statisticians of confusing correlation and causation. He accuses them of mostly steering clear of causal reasoning. I agree with you that his tone is arrogant, but I think he has a point.
– mitchus
Nov 15 at 13:19
|
show 9 more comments
12
I am a non-statistician whose formal training in statistics was by non-statisticians in the same field, and I teach and research with non-statisticians applying statistics. I can assure you that the principle that (e.g.) correlation is not causation is, and was, a recurrent mantra in my field. Indeed I don't come across people who can't see that a correlation between rainfall and wheat yield isn't all that needs to be said about the relationship between them and the underlying processes. Typically, in my experience, non-statisticians too have thought this through long since.
– Nick Cox
Nov 14 at 12:49
7
As an epidemiologist, I'm getting more and more annoyed by this mantra. As @NickCox says, this is something that even non-scientists understand. The problem I have is when everyone jumps on the bandwagon of "correlation does not mean causation!" whenever an observational study (a case-control study, say) is published. Yes, correlation does not mean causation but the researchers are usually quite aware of that and will do everything to design and analyze a study in such a way that a causal interpretation is at least plausible.
– COOLSerdash
Nov 14 at 12:59
4
@Nick Cox: I have edited to more accurately state that there are many non-statisticians who understand this well. It was not my intention to cast dispersions over other professions - only to stress that the issue is extremely well understood by statisticians.
– Ben
Nov 14 at 13:00
7
@NickCox There's a lot more to Pearl's contributions about causality than "correlation is not causation". I'm with Carlos here. There's enough to learn about causality that it should be a whole course. As far as I know, most statistics departments don't offer such a course.
– Neil G
Nov 15 at 0:35
11
@Ben : Pearl does not accuse statisticians of confusing correlation and causation. He accuses them of mostly steering clear of causal reasoning. I agree with you that his tone is arrogant, but I think he has a point.
– mitchus
Nov 15 at 13:19
12
12
I am a non-statistician whose formal training in statistics was by non-statisticians in the same field, and I teach and research with non-statisticians applying statistics. I can assure you that the principle that (e.g.) correlation is not causation is, and was, a recurrent mantra in my field. Indeed I don't come across people who can't see that a correlation between rainfall and wheat yield isn't all that needs to be said about the relationship between them and the underlying processes. Typically, in my experience, non-statisticians too have thought this through long since.
– Nick Cox
Nov 14 at 12:49
I am a non-statistician whose formal training in statistics was by non-statisticians in the same field, and I teach and research with non-statisticians applying statistics. I can assure you that the principle that (e.g.) correlation is not causation is, and was, a recurrent mantra in my field. Indeed I don't come across people who can't see that a correlation between rainfall and wheat yield isn't all that needs to be said about the relationship between them and the underlying processes. Typically, in my experience, non-statisticians too have thought this through long since.
– Nick Cox
Nov 14 at 12:49
7
7
As an epidemiologist, I'm getting more and more annoyed by this mantra. As @NickCox says, this is something that even non-scientists understand. The problem I have is when everyone jumps on the bandwagon of "correlation does not mean causation!" whenever an observational study (a case-control study, say) is published. Yes, correlation does not mean causation but the researchers are usually quite aware of that and will do everything to design and analyze a study in such a way that a causal interpretation is at least plausible.
– COOLSerdash
Nov 14 at 12:59
As an epidemiologist, I'm getting more and more annoyed by this mantra. As @NickCox says, this is something that even non-scientists understand. The problem I have is when everyone jumps on the bandwagon of "correlation does not mean causation!" whenever an observational study (a case-control study, say) is published. Yes, correlation does not mean causation but the researchers are usually quite aware of that and will do everything to design and analyze a study in such a way that a causal interpretation is at least plausible.
– COOLSerdash
Nov 14 at 12:59
4
4
@Nick Cox: I have edited to more accurately state that there are many non-statisticians who understand this well. It was not my intention to cast dispersions over other professions - only to stress that the issue is extremely well understood by statisticians.
– Ben
Nov 14 at 13:00
@Nick Cox: I have edited to more accurately state that there are many non-statisticians who understand this well. It was not my intention to cast dispersions over other professions - only to stress that the issue is extremely well understood by statisticians.
– Ben
Nov 14 at 13:00
7
7
@NickCox There's a lot more to Pearl's contributions about causality than "correlation is not causation". I'm with Carlos here. There's enough to learn about causality that it should be a whole course. As far as I know, most statistics departments don't offer such a course.
– Neil G
Nov 15 at 0:35
@NickCox There's a lot more to Pearl's contributions about causality than "correlation is not causation". I'm with Carlos here. There's enough to learn about causality that it should be a whole course. As far as I know, most statistics departments don't offer such a course.
– Neil G
Nov 15 at 0:35
11
11
@Ben : Pearl does not accuse statisticians of confusing correlation and causation. He accuses them of mostly steering clear of causal reasoning. I agree with you that his tone is arrogant, but I think he has a point.
– mitchus
Nov 15 at 13:19
@Ben : Pearl does not accuse statisticians of confusing correlation and causation. He accuses them of mostly steering clear of causal reasoning. I agree with you that his tone is arrogant, but I think he has a point.
– mitchus
Nov 15 at 13:19
|
show 9 more comments
up vote
8
down vote
a simple linear regression is essentially a causal model
Here's an example I came up with where a linear regression model fails to be causal. Let's say a priori that a drug was taken at time 0 (t=0) and that it has no effect on the rate of heart attacks at t=1. Heart attacks at t=1 affect heart attacks at t=2 (i.e. previous damage makes the heart more susceptible to damage). Survival at t=3 only depends on whether or not people had a heart attack at t=2 -- heart attack at t=1 realistically would affect survival at t=3, but we won't have an arrow, for the sake of simplicity.
Here's the legend:
Here's the true causal graph:
Let's pretend that we don't know that heart attacks at t=1 are independent of taking the drug at t=0 so we construct a simple linear regression model to estimate the effect of the drug on heart attack at t=0. Here our predictor would be Drug t=0 and our outcome variable would be Heart Attack t=1. The only data we have is people who survive at t=3, so we'll run our regression on that data.
Here's the 95% Bayesian credible interval for coefficient of Drug t=0:
Much of the probability as we can see is greater than 0, so it looks like there's an effect! However, we know a priori that there is 0 effect. The mathematics of causation as developed by Judea Pearl and others make it much easier to see that there will be bias in this example (due to conditioning on a descendant of a collider). Judea's work implies that in this situation, we should use the full data set (i.e. don't look at the people who only survived), which will remove the biased paths:
Here's the 95% Credible Interval when looking at the full data set (i.e. not conditioning on those who survived).
.
It is densely centered at 0, which essentially shows no association at all.
In real-life examples, things might not be so simple. There may be many more variables that might cause systematic bias (confounding, selection bias, etc.). What to adjust for in analyses has been mathematized by Pearl; algorithms can suggest which variable to adjust for, or even tell us when adjusting is not enough to remove systematic bias. With this formal theory set in place, we don't need to spend so much time arguing about what to adjust for and what not to adjust for; we can quickly reach conclusions as to whether or not our results are sound. We can design our experiments better, we can analyze observational data more easily.
Here's a freely-available course online on Causal DAGs by Miguel Hernàn. It has a bunch of real-life case studies where professors / scientists / statisticians have come to opposite conclusions about the question at hand. Some of them might seem like paradoxes. However, you can easily solve them via Judea Pearl's d-separation and backdoor-criterion.
For reference, here's code to the data-generating process and the code for credible intervals shown above:
import numpy as np
import pandas as pd
import statsmodels as sm
import pymc3 as pm
from sklearn.linear_model import LinearRegression
%matplotlib inline
# notice that taking the drug is independent of heart attack at time 1.
# heart_attack_time_1 doesn't "listen" to take_drug_t_0
take_drug_t_0 = np.random.binomial(n=1, p=0.7, size=10000)
heart_attack_time_1 = np.random.binomial(n=1, p=0.4, size=10000)
proba_heart_attack_time_2 =
# heart_attack_time_1 increases the probability of heart_attack_time_2. Let's say
# it's because it weakens the heart and makes it more susceptible to further
# injuries
#
# Yet, take_drug_t_0 decreases the probability of heart attacks happening at
# time 2
for drug_t_0, heart_attack_t_1 in zip(take_drug_t_0, heart_attack_time_1):
if drug_t_0 == 0 and heart_attack_t_1 == 0:
proba_heart_attack_time_2.append(0.1)
elif drug_t_0 == 1 and heart_attack_t_1 == 0:
proba_heart_attack_time_2.append(0.1)
elif drug_t_0 == 0 and heart_attack_t_1 == 1:
proba_heart_attack_time_2.append(0.5)
elif drug_t_0 == 1 and heart_attack_t_1 == 1:
proba_heart_attack_time_2.append(0.05)
heart_attack_time_2 = np.random.binomial(
n=2, p=proba_heart_attack_time_2, size=10000
)
# people who've had a heart attack at time 2 are more likely to die by time 3
proba_survive_t_3 =
for heart_attack_t_2 in heart_attack_time_2:
if heart_attack_t_2 == 0:
proba_survive_t_3.append(0.95)
else:
proba_survive_t_3.append(0.6)
survive_t_3 = np.random.binomial(
n=1, p=proba_survive_t_3, size=10000
)
df = pd.DataFrame(
{
'survive_t_3': survive_t_3,
'take_drug_t_0': take_drug_t_0,
'heart_attack_time_1': heart_attack_time_1,
'heart_attack_time_2': heart_attack_time_2
}
)
# we only have access to data of the people who survived
survive_t_3_data = df[
df['survive_t_3'] == 1
]
survive_t_3_X = survive_t_3_data[['take_drug_t_0']]
lr = LinearRegression()
lr.fit(survive_t_3_X, survive_t_3_data['heart_attack_time_1'])
lr.coef_
with pm.Model() as collider_bias_model_normal:
alpha = pm.Normal(name='alpha', mu=0, sd=1)
take_drug_t_0 = pm.Normal(name='take_drug_t_0', mu=0, sd=1)
summation = alpha + take_drug_t_0 * survive_t_3_data['take_drug_t_0']
sigma = pm.Exponential('sigma', lam=1)
pm.Normal(
name='observed',
mu=summation,
sd=sigma,
observed=survive_t_3_data['heart_attack_time_1']
)
collider_bias_normal_trace = pm.sample(2000, tune=1000)
pm.plot_posterior(collider_bias_normal_trace['take_drug_t_0'])
with pm.Model() as no_collider_bias_model_normal:
alpha = pm.Normal(name='alpha', mu=0, sd=1)
take_drug_t_0 = pm.Normal(name='take_drug_t_0', mu=0, sd=1)
summation = alpha + take_drug_t_0 * df['take_drug_t_0']
sigma = pm.Exponential('sigma', lam=1)
pm.Normal(
name='observed',
mu=summation,
sd=sigma,
observed=df['heart_attack_time_1']
)
no_collider_bias_normal_trace = pm.sample(2000, tune=2000)
pm.plot_posterior(no_collider_bias_normal_trace['take_drug_t_0'])
New contributor
edderic is a new contributor to this site. Take care in asking for clarification, commenting, and answering.
Check out our Code of Conduct.
add a comment |
up vote
8
down vote
a simple linear regression is essentially a causal model
Here's an example I came up with where a linear regression model fails to be causal. Let's say a priori that a drug was taken at time 0 (t=0) and that it has no effect on the rate of heart attacks at t=1. Heart attacks at t=1 affect heart attacks at t=2 (i.e. previous damage makes the heart more susceptible to damage). Survival at t=3 only depends on whether or not people had a heart attack at t=2 -- heart attack at t=1 realistically would affect survival at t=3, but we won't have an arrow, for the sake of simplicity.
Here's the legend:
Here's the true causal graph:
Let's pretend that we don't know that heart attacks at t=1 are independent of taking the drug at t=0 so we construct a simple linear regression model to estimate the effect of the drug on heart attack at t=0. Here our predictor would be Drug t=0 and our outcome variable would be Heart Attack t=1. The only data we have is people who survive at t=3, so we'll run our regression on that data.
Here's the 95% Bayesian credible interval for coefficient of Drug t=0:
Much of the probability as we can see is greater than 0, so it looks like there's an effect! However, we know a priori that there is 0 effect. The mathematics of causation as developed by Judea Pearl and others make it much easier to see that there will be bias in this example (due to conditioning on a descendant of a collider). Judea's work implies that in this situation, we should use the full data set (i.e. don't look at the people who only survived), which will remove the biased paths:
Here's the 95% Credible Interval when looking at the full data set (i.e. not conditioning on those who survived).
.
It is densely centered at 0, which essentially shows no association at all.
In real-life examples, things might not be so simple. There may be many more variables that might cause systematic bias (confounding, selection bias, etc.). What to adjust for in analyses has been mathematized by Pearl; algorithms can suggest which variable to adjust for, or even tell us when adjusting is not enough to remove systematic bias. With this formal theory set in place, we don't need to spend so much time arguing about what to adjust for and what not to adjust for; we can quickly reach conclusions as to whether or not our results are sound. We can design our experiments better, we can analyze observational data more easily.
Here's a freely-available course online on Causal DAGs by Miguel Hernàn. It has a bunch of real-life case studies where professors / scientists / statisticians have come to opposite conclusions about the question at hand. Some of them might seem like paradoxes. However, you can easily solve them via Judea Pearl's d-separation and backdoor-criterion.
For reference, here's code to the data-generating process and the code for credible intervals shown above:
import numpy as np
import pandas as pd
import statsmodels as sm
import pymc3 as pm
from sklearn.linear_model import LinearRegression
%matplotlib inline
# notice that taking the drug is independent of heart attack at time 1.
# heart_attack_time_1 doesn't "listen" to take_drug_t_0
take_drug_t_0 = np.random.binomial(n=1, p=0.7, size=10000)
heart_attack_time_1 = np.random.binomial(n=1, p=0.4, size=10000)
proba_heart_attack_time_2 =
# heart_attack_time_1 increases the probability of heart_attack_time_2. Let's say
# it's because it weakens the heart and makes it more susceptible to further
# injuries
#
# Yet, take_drug_t_0 decreases the probability of heart attacks happening at
# time 2
for drug_t_0, heart_attack_t_1 in zip(take_drug_t_0, heart_attack_time_1):
if drug_t_0 == 0 and heart_attack_t_1 == 0:
proba_heart_attack_time_2.append(0.1)
elif drug_t_0 == 1 and heart_attack_t_1 == 0:
proba_heart_attack_time_2.append(0.1)
elif drug_t_0 == 0 and heart_attack_t_1 == 1:
proba_heart_attack_time_2.append(0.5)
elif drug_t_0 == 1 and heart_attack_t_1 == 1:
proba_heart_attack_time_2.append(0.05)
heart_attack_time_2 = np.random.binomial(
n=2, p=proba_heart_attack_time_2, size=10000
)
# people who've had a heart attack at time 2 are more likely to die by time 3
proba_survive_t_3 =
for heart_attack_t_2 in heart_attack_time_2:
if heart_attack_t_2 == 0:
proba_survive_t_3.append(0.95)
else:
proba_survive_t_3.append(0.6)
survive_t_3 = np.random.binomial(
n=1, p=proba_survive_t_3, size=10000
)
df = pd.DataFrame(
{
'survive_t_3': survive_t_3,
'take_drug_t_0': take_drug_t_0,
'heart_attack_time_1': heart_attack_time_1,
'heart_attack_time_2': heart_attack_time_2
}
)
# we only have access to data of the people who survived
survive_t_3_data = df[
df['survive_t_3'] == 1
]
survive_t_3_X = survive_t_3_data[['take_drug_t_0']]
lr = LinearRegression()
lr.fit(survive_t_3_X, survive_t_3_data['heart_attack_time_1'])
lr.coef_
with pm.Model() as collider_bias_model_normal:
alpha = pm.Normal(name='alpha', mu=0, sd=1)
take_drug_t_0 = pm.Normal(name='take_drug_t_0', mu=0, sd=1)
summation = alpha + take_drug_t_0 * survive_t_3_data['take_drug_t_0']
sigma = pm.Exponential('sigma', lam=1)
pm.Normal(
name='observed',
mu=summation,
sd=sigma,
observed=survive_t_3_data['heart_attack_time_1']
)
collider_bias_normal_trace = pm.sample(2000, tune=1000)
pm.plot_posterior(collider_bias_normal_trace['take_drug_t_0'])
with pm.Model() as no_collider_bias_model_normal:
alpha = pm.Normal(name='alpha', mu=0, sd=1)
take_drug_t_0 = pm.Normal(name='take_drug_t_0', mu=0, sd=1)
summation = alpha + take_drug_t_0 * df['take_drug_t_0']
sigma = pm.Exponential('sigma', lam=1)
pm.Normal(
name='observed',
mu=summation,
sd=sigma,
observed=df['heart_attack_time_1']
)
no_collider_bias_normal_trace = pm.sample(2000, tune=2000)
pm.plot_posterior(no_collider_bias_normal_trace['take_drug_t_0'])
New contributor
edderic is a new contributor to this site. Take care in asking for clarification, commenting, and answering.
Check out our Code of Conduct.
add a comment |
up vote
8
down vote
up vote
8
down vote
a simple linear regression is essentially a causal model
Here's an example I came up with where a linear regression model fails to be causal. Let's say a priori that a drug was taken at time 0 (t=0) and that it has no effect on the rate of heart attacks at t=1. Heart attacks at t=1 affect heart attacks at t=2 (i.e. previous damage makes the heart more susceptible to damage). Survival at t=3 only depends on whether or not people had a heart attack at t=2 -- heart attack at t=1 realistically would affect survival at t=3, but we won't have an arrow, for the sake of simplicity.
Here's the legend:
Here's the true causal graph:
Let's pretend that we don't know that heart attacks at t=1 are independent of taking the drug at t=0 so we construct a simple linear regression model to estimate the effect of the drug on heart attack at t=0. Here our predictor would be Drug t=0 and our outcome variable would be Heart Attack t=1. The only data we have is people who survive at t=3, so we'll run our regression on that data.
Here's the 95% Bayesian credible interval for coefficient of Drug t=0:
Much of the probability as we can see is greater than 0, so it looks like there's an effect! However, we know a priori that there is 0 effect. The mathematics of causation as developed by Judea Pearl and others make it much easier to see that there will be bias in this example (due to conditioning on a descendant of a collider). Judea's work implies that in this situation, we should use the full data set (i.e. don't look at the people who only survived), which will remove the biased paths:
Here's the 95% Credible Interval when looking at the full data set (i.e. not conditioning on those who survived).
.
It is densely centered at 0, which essentially shows no association at all.
In real-life examples, things might not be so simple. There may be many more variables that might cause systematic bias (confounding, selection bias, etc.). What to adjust for in analyses has been mathematized by Pearl; algorithms can suggest which variable to adjust for, or even tell us when adjusting is not enough to remove systematic bias. With this formal theory set in place, we don't need to spend so much time arguing about what to adjust for and what not to adjust for; we can quickly reach conclusions as to whether or not our results are sound. We can design our experiments better, we can analyze observational data more easily.
Here's a freely-available course online on Causal DAGs by Miguel Hernàn. It has a bunch of real-life case studies where professors / scientists / statisticians have come to opposite conclusions about the question at hand. Some of them might seem like paradoxes. However, you can easily solve them via Judea Pearl's d-separation and backdoor-criterion.
For reference, here's code to the data-generating process and the code for credible intervals shown above:
import numpy as np
import pandas as pd
import statsmodels as sm
import pymc3 as pm
from sklearn.linear_model import LinearRegression
%matplotlib inline
# notice that taking the drug is independent of heart attack at time 1.
# heart_attack_time_1 doesn't "listen" to take_drug_t_0
take_drug_t_0 = np.random.binomial(n=1, p=0.7, size=10000)
heart_attack_time_1 = np.random.binomial(n=1, p=0.4, size=10000)
proba_heart_attack_time_2 =
# heart_attack_time_1 increases the probability of heart_attack_time_2. Let's say
# it's because it weakens the heart and makes it more susceptible to further
# injuries
#
# Yet, take_drug_t_0 decreases the probability of heart attacks happening at
# time 2
for drug_t_0, heart_attack_t_1 in zip(take_drug_t_0, heart_attack_time_1):
if drug_t_0 == 0 and heart_attack_t_1 == 0:
proba_heart_attack_time_2.append(0.1)
elif drug_t_0 == 1 and heart_attack_t_1 == 0:
proba_heart_attack_time_2.append(0.1)
elif drug_t_0 == 0 and heart_attack_t_1 == 1:
proba_heart_attack_time_2.append(0.5)
elif drug_t_0 == 1 and heart_attack_t_1 == 1:
proba_heart_attack_time_2.append(0.05)
heart_attack_time_2 = np.random.binomial(
n=2, p=proba_heart_attack_time_2, size=10000
)
# people who've had a heart attack at time 2 are more likely to die by time 3
proba_survive_t_3 =
for heart_attack_t_2 in heart_attack_time_2:
if heart_attack_t_2 == 0:
proba_survive_t_3.append(0.95)
else:
proba_survive_t_3.append(0.6)
survive_t_3 = np.random.binomial(
n=1, p=proba_survive_t_3, size=10000
)
df = pd.DataFrame(
{
'survive_t_3': survive_t_3,
'take_drug_t_0': take_drug_t_0,
'heart_attack_time_1': heart_attack_time_1,
'heart_attack_time_2': heart_attack_time_2
}
)
# we only have access to data of the people who survived
survive_t_3_data = df[
df['survive_t_3'] == 1
]
survive_t_3_X = survive_t_3_data[['take_drug_t_0']]
lr = LinearRegression()
lr.fit(survive_t_3_X, survive_t_3_data['heart_attack_time_1'])
lr.coef_
with pm.Model() as collider_bias_model_normal:
alpha = pm.Normal(name='alpha', mu=0, sd=1)
take_drug_t_0 = pm.Normal(name='take_drug_t_0', mu=0, sd=1)
summation = alpha + take_drug_t_0 * survive_t_3_data['take_drug_t_0']
sigma = pm.Exponential('sigma', lam=1)
pm.Normal(
name='observed',
mu=summation,
sd=sigma,
observed=survive_t_3_data['heart_attack_time_1']
)
collider_bias_normal_trace = pm.sample(2000, tune=1000)
pm.plot_posterior(collider_bias_normal_trace['take_drug_t_0'])
with pm.Model() as no_collider_bias_model_normal:
alpha = pm.Normal(name='alpha', mu=0, sd=1)
take_drug_t_0 = pm.Normal(name='take_drug_t_0', mu=0, sd=1)
summation = alpha + take_drug_t_0 * df['take_drug_t_0']
sigma = pm.Exponential('sigma', lam=1)
pm.Normal(
name='observed',
mu=summation,
sd=sigma,
observed=df['heart_attack_time_1']
)
no_collider_bias_normal_trace = pm.sample(2000, tune=2000)
pm.plot_posterior(no_collider_bias_normal_trace['take_drug_t_0'])
New contributor
edderic is a new contributor to this site. Take care in asking for clarification, commenting, and answering.
Check out our Code of Conduct.
a simple linear regression is essentially a causal model
Here's an example I came up with where a linear regression model fails to be causal. Let's say a priori that a drug was taken at time 0 (t=0) and that it has no effect on the rate of heart attacks at t=1. Heart attacks at t=1 affect heart attacks at t=2 (i.e. previous damage makes the heart more susceptible to damage). Survival at t=3 only depends on whether or not people had a heart attack at t=2 -- heart attack at t=1 realistically would affect survival at t=3, but we won't have an arrow, for the sake of simplicity.
Here's the legend:
Here's the true causal graph:
Let's pretend that we don't know that heart attacks at t=1 are independent of taking the drug at t=0 so we construct a simple linear regression model to estimate the effect of the drug on heart attack at t=0. Here our predictor would be Drug t=0 and our outcome variable would be Heart Attack t=1. The only data we have is people who survive at t=3, so we'll run our regression on that data.
Here's the 95% Bayesian credible interval for coefficient of Drug t=0:
Much of the probability as we can see is greater than 0, so it looks like there's an effect! However, we know a priori that there is 0 effect. The mathematics of causation as developed by Judea Pearl and others make it much easier to see that there will be bias in this example (due to conditioning on a descendant of a collider). Judea's work implies that in this situation, we should use the full data set (i.e. don't look at the people who only survived), which will remove the biased paths:
Here's the 95% Credible Interval when looking at the full data set (i.e. not conditioning on those who survived).
.
It is densely centered at 0, which essentially shows no association at all.
In real-life examples, things might not be so simple. There may be many more variables that might cause systematic bias (confounding, selection bias, etc.). What to adjust for in analyses has been mathematized by Pearl; algorithms can suggest which variable to adjust for, or even tell us when adjusting is not enough to remove systematic bias. With this formal theory set in place, we don't need to spend so much time arguing about what to adjust for and what not to adjust for; we can quickly reach conclusions as to whether or not our results are sound. We can design our experiments better, we can analyze observational data more easily.
Here's a freely-available course online on Causal DAGs by Miguel Hernàn. It has a bunch of real-life case studies where professors / scientists / statisticians have come to opposite conclusions about the question at hand. Some of them might seem like paradoxes. However, you can easily solve them via Judea Pearl's d-separation and backdoor-criterion.
For reference, here's code to the data-generating process and the code for credible intervals shown above:
import numpy as np
import pandas as pd
import statsmodels as sm
import pymc3 as pm
from sklearn.linear_model import LinearRegression
%matplotlib inline
# notice that taking the drug is independent of heart attack at time 1.
# heart_attack_time_1 doesn't "listen" to take_drug_t_0
take_drug_t_0 = np.random.binomial(n=1, p=0.7, size=10000)
heart_attack_time_1 = np.random.binomial(n=1, p=0.4, size=10000)
proba_heart_attack_time_2 =
# heart_attack_time_1 increases the probability of heart_attack_time_2. Let's say
# it's because it weakens the heart and makes it more susceptible to further
# injuries
#
# Yet, take_drug_t_0 decreases the probability of heart attacks happening at
# time 2
for drug_t_0, heart_attack_t_1 in zip(take_drug_t_0, heart_attack_time_1):
if drug_t_0 == 0 and heart_attack_t_1 == 0:
proba_heart_attack_time_2.append(0.1)
elif drug_t_0 == 1 and heart_attack_t_1 == 0:
proba_heart_attack_time_2.append(0.1)
elif drug_t_0 == 0 and heart_attack_t_1 == 1:
proba_heart_attack_time_2.append(0.5)
elif drug_t_0 == 1 and heart_attack_t_1 == 1:
proba_heart_attack_time_2.append(0.05)
heart_attack_time_2 = np.random.binomial(
n=2, p=proba_heart_attack_time_2, size=10000
)
# people who've had a heart attack at time 2 are more likely to die by time 3
proba_survive_t_3 =
for heart_attack_t_2 in heart_attack_time_2:
if heart_attack_t_2 == 0:
proba_survive_t_3.append(0.95)
else:
proba_survive_t_3.append(0.6)
survive_t_3 = np.random.binomial(
n=1, p=proba_survive_t_3, size=10000
)
df = pd.DataFrame(
{
'survive_t_3': survive_t_3,
'take_drug_t_0': take_drug_t_0,
'heart_attack_time_1': heart_attack_time_1,
'heart_attack_time_2': heart_attack_time_2
}
)
# we only have access to data of the people who survived
survive_t_3_data = df[
df['survive_t_3'] == 1
]
survive_t_3_X = survive_t_3_data[['take_drug_t_0']]
lr = LinearRegression()
lr.fit(survive_t_3_X, survive_t_3_data['heart_attack_time_1'])
lr.coef_
with pm.Model() as collider_bias_model_normal:
alpha = pm.Normal(name='alpha', mu=0, sd=1)
take_drug_t_0 = pm.Normal(name='take_drug_t_0', mu=0, sd=1)
summation = alpha + take_drug_t_0 * survive_t_3_data['take_drug_t_0']
sigma = pm.Exponential('sigma', lam=1)
pm.Normal(
name='observed',
mu=summation,
sd=sigma,
observed=survive_t_3_data['heart_attack_time_1']
)
collider_bias_normal_trace = pm.sample(2000, tune=1000)
pm.plot_posterior(collider_bias_normal_trace['take_drug_t_0'])
with pm.Model() as no_collider_bias_model_normal:
alpha = pm.Normal(name='alpha', mu=0, sd=1)
take_drug_t_0 = pm.Normal(name='take_drug_t_0', mu=0, sd=1)
summation = alpha + take_drug_t_0 * df['take_drug_t_0']
sigma = pm.Exponential('sigma', lam=1)
pm.Normal(
name='observed',
mu=summation,
sd=sigma,
observed=df['heart_attack_time_1']
)
no_collider_bias_normal_trace = pm.sample(2000, tune=2000)
pm.plot_posterior(no_collider_bias_normal_trace['take_drug_t_0'])
New contributor
edderic is a new contributor to this site. Take care in asking for clarification, commenting, and answering.
Check out our Code of Conduct.
New contributor
edderic is a new contributor to this site. Take care in asking for clarification, commenting, and answering.
Check out our Code of Conduct.
answered Nov 15 at 16:14
edderic
814
814
New contributor
edderic is a new contributor to this site. Take care in asking for clarification, commenting, and answering.
Check out our Code of Conduct.
New contributor
edderic is a new contributor to this site. Take care in asking for clarification, commenting, and answering.
Check out our Code of Conduct.
edderic is a new contributor to this site. Take care in asking for clarification, commenting, and answering.
Check out our Code of Conduct.
add a comment |
add a comment |
up vote
4
down vote
Two papers, the second a classic, that help (I think) shed additional lights on Judea's points and this topic more generally. This comes from someone who has used SEM (which is correlation and regression) repeatedly and resonates with his critiques:
https://www.sciencedirect.com/science/article/pii/S0022103111001466
http://psycnet.apa.org/record/1973-20037-001
Essentially the papers describe why correlational models (regression) can not ordinarily be taken as implying any strong causal inference. Any pattern of associations can fit a given covariance matrix (i.e., non specification of direction and or relationship among the variables). Hence the need for such things as an experimental design, counterfactual propositions, etc. This even applies when one has a temporal structure to their data where the putative cause occurs in time before the putative effect.
add a comment |
up vote
4
down vote
Two papers, the second a classic, that help (I think) shed additional lights on Judea's points and this topic more generally. This comes from someone who has used SEM (which is correlation and regression) repeatedly and resonates with his critiques:
https://www.sciencedirect.com/science/article/pii/S0022103111001466
http://psycnet.apa.org/record/1973-20037-001
Essentially the papers describe why correlational models (regression) can not ordinarily be taken as implying any strong causal inference. Any pattern of associations can fit a given covariance matrix (i.e., non specification of direction and or relationship among the variables). Hence the need for such things as an experimental design, counterfactual propositions, etc. This even applies when one has a temporal structure to their data where the putative cause occurs in time before the putative effect.
add a comment |
up vote
4
down vote
up vote
4
down vote
Two papers, the second a classic, that help (I think) shed additional lights on Judea's points and this topic more generally. This comes from someone who has used SEM (which is correlation and regression) repeatedly and resonates with his critiques:
https://www.sciencedirect.com/science/article/pii/S0022103111001466
http://psycnet.apa.org/record/1973-20037-001
Essentially the papers describe why correlational models (regression) can not ordinarily be taken as implying any strong causal inference. Any pattern of associations can fit a given covariance matrix (i.e., non specification of direction and or relationship among the variables). Hence the need for such things as an experimental design, counterfactual propositions, etc. This even applies when one has a temporal structure to their data where the putative cause occurs in time before the putative effect.
Two papers, the second a classic, that help (I think) shed additional lights on Judea's points and this topic more generally. This comes from someone who has used SEM (which is correlation and regression) repeatedly and resonates with his critiques:
https://www.sciencedirect.com/science/article/pii/S0022103111001466
http://psycnet.apa.org/record/1973-20037-001
Essentially the papers describe why correlational models (regression) can not ordinarily be taken as implying any strong causal inference. Any pattern of associations can fit a given covariance matrix (i.e., non specification of direction and or relationship among the variables). Hence the need for such things as an experimental design, counterfactual propositions, etc. This even applies when one has a temporal structure to their data where the putative cause occurs in time before the putative effect.
edited Nov 15 at 22:15
answered Nov 15 at 10:38


Jhaltiga68
12319
12319
add a comment |
add a comment |
up vote
1
down vote
"...since we are essentially assuming that one variable is the cause and another is the effect (hence correlation is different approach from regression modelling)..."
Regression modeling most definitely does NOT make this assumption.
"... and testing whether this causal relationship explains the observed patterns."
If you are assuming causality and validating it against observations, your are doing SEM modeling, or what Pearl would call SCM modeling. Whether or not you want to call that part of the domain of stats is debatable. But I think most wouldn't call it classical stats.
Rather than dumping on stats in general, I believe Pearl is just criticizing statistician's reticence to address causal semantics. He considers this a serious problem because of what Carl Sagan calls the "get in and get out" phenomenon, where you drop a study that says "meat consumption 'strongly associated' with increased libido, p < .05" and then bows out knowing full well the two outcomes are going to be causally linked in the mind of the public.
add a comment |
up vote
1
down vote
"...since we are essentially assuming that one variable is the cause and another is the effect (hence correlation is different approach from regression modelling)..."
Regression modeling most definitely does NOT make this assumption.
"... and testing whether this causal relationship explains the observed patterns."
If you are assuming causality and validating it against observations, your are doing SEM modeling, or what Pearl would call SCM modeling. Whether or not you want to call that part of the domain of stats is debatable. But I think most wouldn't call it classical stats.
Rather than dumping on stats in general, I believe Pearl is just criticizing statistician's reticence to address causal semantics. He considers this a serious problem because of what Carl Sagan calls the "get in and get out" phenomenon, where you drop a study that says "meat consumption 'strongly associated' with increased libido, p < .05" and then bows out knowing full well the two outcomes are going to be causally linked in the mind of the public.
add a comment |
up vote
1
down vote
up vote
1
down vote
"...since we are essentially assuming that one variable is the cause and another is the effect (hence correlation is different approach from regression modelling)..."
Regression modeling most definitely does NOT make this assumption.
"... and testing whether this causal relationship explains the observed patterns."
If you are assuming causality and validating it against observations, your are doing SEM modeling, or what Pearl would call SCM modeling. Whether or not you want to call that part of the domain of stats is debatable. But I think most wouldn't call it classical stats.
Rather than dumping on stats in general, I believe Pearl is just criticizing statistician's reticence to address causal semantics. He considers this a serious problem because of what Carl Sagan calls the "get in and get out" phenomenon, where you drop a study that says "meat consumption 'strongly associated' with increased libido, p < .05" and then bows out knowing full well the two outcomes are going to be causally linked in the mind of the public.
"...since we are essentially assuming that one variable is the cause and another is the effect (hence correlation is different approach from regression modelling)..."
Regression modeling most definitely does NOT make this assumption.
"... and testing whether this causal relationship explains the observed patterns."
If you are assuming causality and validating it against observations, your are doing SEM modeling, or what Pearl would call SCM modeling. Whether or not you want to call that part of the domain of stats is debatable. But I think most wouldn't call it classical stats.
Rather than dumping on stats in general, I believe Pearl is just criticizing statistician's reticence to address causal semantics. He considers this a serious problem because of what Carl Sagan calls the "get in and get out" phenomenon, where you drop a study that says "meat consumption 'strongly associated' with increased libido, p < .05" and then bows out knowing full well the two outcomes are going to be causally linked in the mind of the public.
answered Nov 15 at 23:39
Count Zero
424211
424211
add a comment |
add a comment |
Sign up or log in
StackExchange.ready(function () {
StackExchange.helpers.onClickDraftSave('#login-link');
});
Sign up using Google
Sign up using Facebook
Sign up using Email and Password
Post as a guest
Required, but never shown
StackExchange.ready(
function () {
StackExchange.openid.initPostLogin('.new-post-login', 'https%3a%2f%2fstats.stackexchange.com%2fquestions%2f376920%2fthe-book-of-why-by-judea-pearl-why-is-he-bashing-statistics%23new-answer', 'question_page');
}
);
Post as a guest
Required, but never shown
Sign up or log in
StackExchange.ready(function () {
StackExchange.helpers.onClickDraftSave('#login-link');
});
Sign up using Google
Sign up using Facebook
Sign up using Email and Password
Post as a guest
Required, but never shown
Sign up or log in
StackExchange.ready(function () {
StackExchange.helpers.onClickDraftSave('#login-link');
});
Sign up using Google
Sign up using Facebook
Sign up using Email and Password
Post as a guest
Required, but never shown
Sign up or log in
StackExchange.ready(function () {
StackExchange.helpers.onClickDraftSave('#login-link');
});
Sign up using Google
Sign up using Facebook
Sign up using Email and Password
Sign up using Google
Sign up using Facebook
Sign up using Email and Password
Post as a guest
Required, but never shown
Required, but never shown
Required, but never shown
Required, but never shown
Required, but never shown
Required, but never shown
Required, but never shown
Required, but never shown
Required, but never shown
PpATdQCRKwWUhfP9 R9YJVMZ3B6F,fTL8Ge2,U8zESwouhuU,Zrk,p8nyY jFGu,KbOi64Iw,jPKk,FTf3z
35
Linear regression is not a causal model. Simple linear regression is the same as pairwise correlation, the only difference is standarization. So if you say that regression is causal, then same should be true also for correlation. Is correlation causation? You can use regression to predict whatever, nonsense relations between any arbitrary variables (with many "significant" results by chance).
– Tim♦
Nov 14 at 9:35
7
Disagreements over which approach to reasoning about causality in statistics has most merit between Pearl, Rubin, Heckman and others appear to have festered, and I do think Pearl's tone is getting ever haughtier. Don't let that distract you from the genuine insight he has to offer. Read his earlier book Causality, it will get under your skin less.
– CloseToC
Nov 14 at 10:01
6
@CloseToC I would add that Pearl, Rubin and Heckman are in a way all working within the same framework (i.e., logically equivalent frameworks, see here stats.stackexchange.com/questions/249767/…), so their disputes are in a different level from arguing things like "linear regression is a causal model".
– Carlos Cinelli
Nov 14 at 10:04
8
I have been irritated by the book myself. There are some simply false statistical claims there (cannot cite now, the book with my notes in the margins is at home) which made me wonder whether only the journalist who helped Pearl write the book or also Pearl himself was a poor statistician. (Needless to say, I was very surprised to discover such blatant mistakes in a work of such a revered scientist.) His papers are much better, though even there no one would accuse Pearl for modesty...
– Richard Hardy
Nov 14 at 13:32
14
I have some concern that this thread already tangles together (a) a specific book from a very smart person (b) that smart person's persona and style of debate (c) whether a particular viewpoint is correct, exaggerated, or whatever.
– Nick Cox
Nov 14 at 13:41