Corrupted Graph in Keras models when they are converted to TensorFlow graph
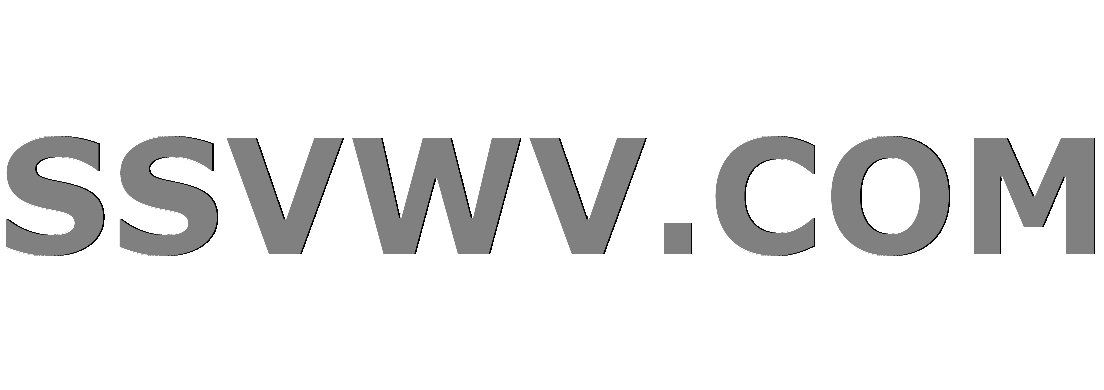
Multi tool use
up vote
0
down vote
favorite
I developed two keras models inside two unit tests. I converted the models into tensorflow graph (using https://github.com/amir-abdi/keras_to_tensorflow) to store on disk. When the tests are run separately, the models are loaded fine and work as they are expected to do. But when I run the test through unittest discover
, I got the following error running the second test:
Tensor dense_2_target:0, specified in either feed_devices or fetch_devices was not found in the Graph
.
I am wondering if it is a cause of any open resources or dependencies between the generated graphs? Any help is appreciated.
Here is the source code for the two models.
Model 1:
model = Sequential(name="Regressor")
model.add(Dense(10, input_dim=2, activation='relu'))
model.add(Dense(10, activation='relu'))
model.add(Dense(1, activation=None))
model.compile(loss='mean_absolute_error', optimizer='adam')
model.fit(X, y, verbose=0)
convert_to_tensorflow_graph() # as described in https://github.com/amir-abdi/keras_to_tensorflow.
Model 2:
model = Sequential(name="classifier")
model.add(Dense(10, input_dim=4, activation='relu'))
model.add(Dense(1, activation='sigmoid'))
model.compile(loss='binary_crossentropy', optimizer='adam')
model.fit(X, y, verbose=0)
convert_to_tensorflow_graph()
As you see, the structure of the models are not the same.
python tensorflow machine-learning keras deep-learning
add a comment |
up vote
0
down vote
favorite
I developed two keras models inside two unit tests. I converted the models into tensorflow graph (using https://github.com/amir-abdi/keras_to_tensorflow) to store on disk. When the tests are run separately, the models are loaded fine and work as they are expected to do. But when I run the test through unittest discover
, I got the following error running the second test:
Tensor dense_2_target:0, specified in either feed_devices or fetch_devices was not found in the Graph
.
I am wondering if it is a cause of any open resources or dependencies between the generated graphs? Any help is appreciated.
Here is the source code for the two models.
Model 1:
model = Sequential(name="Regressor")
model.add(Dense(10, input_dim=2, activation='relu'))
model.add(Dense(10, activation='relu'))
model.add(Dense(1, activation=None))
model.compile(loss='mean_absolute_error', optimizer='adam')
model.fit(X, y, verbose=0)
convert_to_tensorflow_graph() # as described in https://github.com/amir-abdi/keras_to_tensorflow.
Model 2:
model = Sequential(name="classifier")
model.add(Dense(10, input_dim=4, activation='relu'))
model.add(Dense(1, activation='sigmoid'))
model.compile(loss='binary_crossentropy', optimizer='adam')
model.fit(X, y, verbose=0)
convert_to_tensorflow_graph()
As you see, the structure of the models are not the same.
python tensorflow machine-learning keras deep-learning
add a comment |
up vote
0
down vote
favorite
up vote
0
down vote
favorite
I developed two keras models inside two unit tests. I converted the models into tensorflow graph (using https://github.com/amir-abdi/keras_to_tensorflow) to store on disk. When the tests are run separately, the models are loaded fine and work as they are expected to do. But when I run the test through unittest discover
, I got the following error running the second test:
Tensor dense_2_target:0, specified in either feed_devices or fetch_devices was not found in the Graph
.
I am wondering if it is a cause of any open resources or dependencies between the generated graphs? Any help is appreciated.
Here is the source code for the two models.
Model 1:
model = Sequential(name="Regressor")
model.add(Dense(10, input_dim=2, activation='relu'))
model.add(Dense(10, activation='relu'))
model.add(Dense(1, activation=None))
model.compile(loss='mean_absolute_error', optimizer='adam')
model.fit(X, y, verbose=0)
convert_to_tensorflow_graph() # as described in https://github.com/amir-abdi/keras_to_tensorflow.
Model 2:
model = Sequential(name="classifier")
model.add(Dense(10, input_dim=4, activation='relu'))
model.add(Dense(1, activation='sigmoid'))
model.compile(loss='binary_crossentropy', optimizer='adam')
model.fit(X, y, verbose=0)
convert_to_tensorflow_graph()
As you see, the structure of the models are not the same.
python tensorflow machine-learning keras deep-learning
I developed two keras models inside two unit tests. I converted the models into tensorflow graph (using https://github.com/amir-abdi/keras_to_tensorflow) to store on disk. When the tests are run separately, the models are loaded fine and work as they are expected to do. But when I run the test through unittest discover
, I got the following error running the second test:
Tensor dense_2_target:0, specified in either feed_devices or fetch_devices was not found in the Graph
.
I am wondering if it is a cause of any open resources or dependencies between the generated graphs? Any help is appreciated.
Here is the source code for the two models.
Model 1:
model = Sequential(name="Regressor")
model.add(Dense(10, input_dim=2, activation='relu'))
model.add(Dense(10, activation='relu'))
model.add(Dense(1, activation=None))
model.compile(loss='mean_absolute_error', optimizer='adam')
model.fit(X, y, verbose=0)
convert_to_tensorflow_graph() # as described in https://github.com/amir-abdi/keras_to_tensorflow.
Model 2:
model = Sequential(name="classifier")
model.add(Dense(10, input_dim=4, activation='relu'))
model.add(Dense(1, activation='sigmoid'))
model.compile(loss='binary_crossentropy', optimizer='adam')
model.fit(X, y, verbose=0)
convert_to_tensorflow_graph()
As you see, the structure of the models are not the same.
python tensorflow machine-learning keras deep-learning
python tensorflow machine-learning keras deep-learning
edited Nov 20 at 15:59
asked Nov 19 at 18:16


mehdi
468
468
add a comment |
add a comment |
active
oldest
votes
active
oldest
votes
active
oldest
votes
active
oldest
votes
active
oldest
votes
Thanks for contributing an answer to Stack Overflow!
- Please be sure to answer the question. Provide details and share your research!
But avoid …
- Asking for help, clarification, or responding to other answers.
- Making statements based on opinion; back them up with references or personal experience.
To learn more, see our tips on writing great answers.
Some of your past answers have not been well-received, and you're in danger of being blocked from answering.
Please pay close attention to the following guidance:
- Please be sure to answer the question. Provide details and share your research!
But avoid …
- Asking for help, clarification, or responding to other answers.
- Making statements based on opinion; back them up with references or personal experience.
To learn more, see our tips on writing great answers.
Sign up or log in
StackExchange.ready(function () {
StackExchange.helpers.onClickDraftSave('#login-link');
});
Sign up using Google
Sign up using Facebook
Sign up using Email and Password
Post as a guest
Required, but never shown
StackExchange.ready(
function () {
StackExchange.openid.initPostLogin('.new-post-login', 'https%3a%2f%2fstackoverflow.com%2fquestions%2f53380472%2fcorrupted-graph-in-keras-models-when-they-are-converted-to-tensorflow-graph%23new-answer', 'question_page');
}
);
Post as a guest
Required, but never shown
Sign up or log in
StackExchange.ready(function () {
StackExchange.helpers.onClickDraftSave('#login-link');
});
Sign up using Google
Sign up using Facebook
Sign up using Email and Password
Post as a guest
Required, but never shown
Sign up or log in
StackExchange.ready(function () {
StackExchange.helpers.onClickDraftSave('#login-link');
});
Sign up using Google
Sign up using Facebook
Sign up using Email and Password
Post as a guest
Required, but never shown
Sign up or log in
StackExchange.ready(function () {
StackExchange.helpers.onClickDraftSave('#login-link');
});
Sign up using Google
Sign up using Facebook
Sign up using Email and Password
Sign up using Google
Sign up using Facebook
Sign up using Email and Password
Post as a guest
Required, but never shown
Required, but never shown
Required, but never shown
Required, but never shown
Required, but never shown
Required, but never shown
Required, but never shown
Required, but never shown
Required, but never shown
qQMMRE Aix4,r,IJri,ZwFRcptwG