PyTorch set_grad_enabled(False) vs with no_grad():
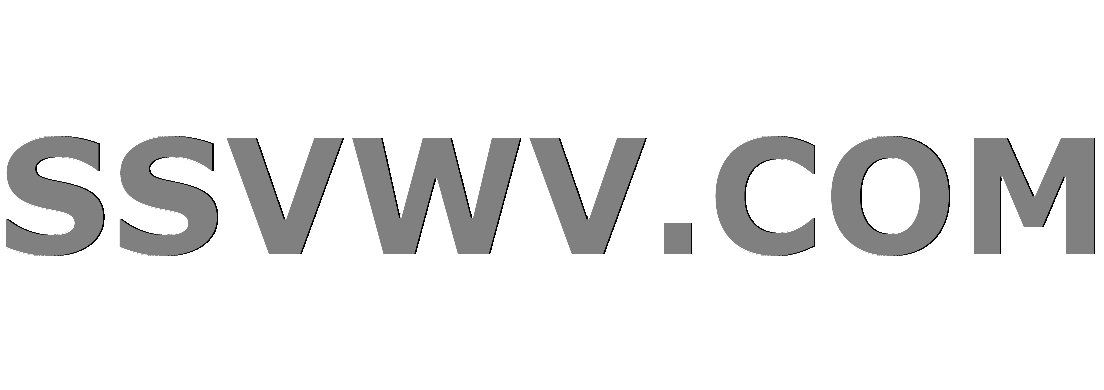
Multi tool use
Assuming autograd is on (as it is by default), is there any difference (besides indent) between doing:
with torch.no_grad():
<code>
and
torch.set_grad_enabled(False)
<code>
torch.set_grad_enabled(True)
pytorch
add a comment |
Assuming autograd is on (as it is by default), is there any difference (besides indent) between doing:
with torch.no_grad():
<code>
and
torch.set_grad_enabled(False)
<code>
torch.set_grad_enabled(True)
pytorch
Related: Save and restore autograd enabled state
– Tom Hale
Nov 23 '18 at 13:15
add a comment |
Assuming autograd is on (as it is by default), is there any difference (besides indent) between doing:
with torch.no_grad():
<code>
and
torch.set_grad_enabled(False)
<code>
torch.set_grad_enabled(True)
pytorch
Assuming autograd is on (as it is by default), is there any difference (besides indent) between doing:
with torch.no_grad():
<code>
and
torch.set_grad_enabled(False)
<code>
torch.set_grad_enabled(True)
pytorch
pytorch
edited Nov 23 '18 at 13:16
Tom Hale
asked Nov 23 '18 at 13:09


Tom HaleTom Hale
7,2314659
7,2314659
Related: Save and restore autograd enabled state
– Tom Hale
Nov 23 '18 at 13:15
add a comment |
Related: Save and restore autograd enabled state
– Tom Hale
Nov 23 '18 at 13:15
Related: Save and restore autograd enabled state
– Tom Hale
Nov 23 '18 at 13:15
Related: Save and restore autograd enabled state
– Tom Hale
Nov 23 '18 at 13:15
add a comment |
1 Answer
1
active
oldest
votes
Actually no, there no difference in the way used in the question. When you take a look at the source code of no_grad
. You see that it is actually using torch.set_grad_enabled
to archive this behaviour:
class no_grad(object):
r"""Context-manager that disabled gradient calculation.
Disabling gradient calculation is useful for inference, when you are sure
that you will not call :meth:`Tensor.backward()`. It will reduce memory
consumption for computations that would otherwise have `requires_grad=True`.
In this mode, the result of every computation will have
`requires_grad=False`, even when the inputs have `requires_grad=True`.
Also functions as a decorator.
Example::
>>> x = torch.tensor([1], requires_grad=True)
>>> with torch.no_grad():
... y = x * 2
>>> y.requires_grad
False
>>> @torch.no_grad()
... def doubler(x):
... return x * 2
>>> z = doubler(x)
>>> z.requires_grad
False
"""
def __init__(self):
self.prev = torch.is_grad_enabled()
def __enter__(self):
torch._C.set_grad_enabled(False)
def __exit__(self, *args):
torch.set_grad_enabled(self.prev)
return False
def __call__(self, func):
@functools.wraps(func)
def decorate_no_grad(*args, **kwargs):
with self:
return func(*args, **kwargs)
return decorate_no_grad
However there is an additional functionality of torch.set_grad_enabled
over torch.no_grad
when used in a with
-statement which lets you control to switch on or off gradient computation:
>>> x = torch.tensor([1], requires_grad=True)
>>> is_train = False
>>> with torch.set_grad_enabled(is_train):
... y = x * 2
>>> y.requires_grad
https://pytorch.org/docs/stable/_modules/torch/autograd/grad_mode.html
add a comment |
Your Answer
StackExchange.ifUsing("editor", function () {
StackExchange.using("externalEditor", function () {
StackExchange.using("snippets", function () {
StackExchange.snippets.init();
});
});
}, "code-snippets");
StackExchange.ready(function() {
var channelOptions = {
tags: "".split(" "),
id: "1"
};
initTagRenderer("".split(" "), "".split(" "), channelOptions);
StackExchange.using("externalEditor", function() {
// Have to fire editor after snippets, if snippets enabled
if (StackExchange.settings.snippets.snippetsEnabled) {
StackExchange.using("snippets", function() {
createEditor();
});
}
else {
createEditor();
}
});
function createEditor() {
StackExchange.prepareEditor({
heartbeatType: 'answer',
autoActivateHeartbeat: false,
convertImagesToLinks: true,
noModals: true,
showLowRepImageUploadWarning: true,
reputationToPostImages: 10,
bindNavPrevention: true,
postfix: "",
imageUploader: {
brandingHtml: "Powered by u003ca class="icon-imgur-white" href="https://imgur.com/"u003eu003c/au003e",
contentPolicyHtml: "User contributions licensed under u003ca href="https://creativecommons.org/licenses/by-sa/3.0/"u003ecc by-sa 3.0 with attribution requiredu003c/au003e u003ca href="https://stackoverflow.com/legal/content-policy"u003e(content policy)u003c/au003e",
allowUrls: true
},
onDemand: true,
discardSelector: ".discard-answer"
,immediatelyShowMarkdownHelp:true
});
}
});
Sign up or log in
StackExchange.ready(function () {
StackExchange.helpers.onClickDraftSave('#login-link');
});
Sign up using Google
Sign up using Facebook
Sign up using Email and Password
Post as a guest
Required, but never shown
StackExchange.ready(
function () {
StackExchange.openid.initPostLogin('.new-post-login', 'https%3a%2f%2fstackoverflow.com%2fquestions%2f53447345%2fpytorch-set-grad-enabledfalse-vs-with-no-grad%23new-answer', 'question_page');
}
);
Post as a guest
Required, but never shown
1 Answer
1
active
oldest
votes
1 Answer
1
active
oldest
votes
active
oldest
votes
active
oldest
votes
Actually no, there no difference in the way used in the question. When you take a look at the source code of no_grad
. You see that it is actually using torch.set_grad_enabled
to archive this behaviour:
class no_grad(object):
r"""Context-manager that disabled gradient calculation.
Disabling gradient calculation is useful for inference, when you are sure
that you will not call :meth:`Tensor.backward()`. It will reduce memory
consumption for computations that would otherwise have `requires_grad=True`.
In this mode, the result of every computation will have
`requires_grad=False`, even when the inputs have `requires_grad=True`.
Also functions as a decorator.
Example::
>>> x = torch.tensor([1], requires_grad=True)
>>> with torch.no_grad():
... y = x * 2
>>> y.requires_grad
False
>>> @torch.no_grad()
... def doubler(x):
... return x * 2
>>> z = doubler(x)
>>> z.requires_grad
False
"""
def __init__(self):
self.prev = torch.is_grad_enabled()
def __enter__(self):
torch._C.set_grad_enabled(False)
def __exit__(self, *args):
torch.set_grad_enabled(self.prev)
return False
def __call__(self, func):
@functools.wraps(func)
def decorate_no_grad(*args, **kwargs):
with self:
return func(*args, **kwargs)
return decorate_no_grad
However there is an additional functionality of torch.set_grad_enabled
over torch.no_grad
when used in a with
-statement which lets you control to switch on or off gradient computation:
>>> x = torch.tensor([1], requires_grad=True)
>>> is_train = False
>>> with torch.set_grad_enabled(is_train):
... y = x * 2
>>> y.requires_grad
https://pytorch.org/docs/stable/_modules/torch/autograd/grad_mode.html
add a comment |
Actually no, there no difference in the way used in the question. When you take a look at the source code of no_grad
. You see that it is actually using torch.set_grad_enabled
to archive this behaviour:
class no_grad(object):
r"""Context-manager that disabled gradient calculation.
Disabling gradient calculation is useful for inference, when you are sure
that you will not call :meth:`Tensor.backward()`. It will reduce memory
consumption for computations that would otherwise have `requires_grad=True`.
In this mode, the result of every computation will have
`requires_grad=False`, even when the inputs have `requires_grad=True`.
Also functions as a decorator.
Example::
>>> x = torch.tensor([1], requires_grad=True)
>>> with torch.no_grad():
... y = x * 2
>>> y.requires_grad
False
>>> @torch.no_grad()
... def doubler(x):
... return x * 2
>>> z = doubler(x)
>>> z.requires_grad
False
"""
def __init__(self):
self.prev = torch.is_grad_enabled()
def __enter__(self):
torch._C.set_grad_enabled(False)
def __exit__(self, *args):
torch.set_grad_enabled(self.prev)
return False
def __call__(self, func):
@functools.wraps(func)
def decorate_no_grad(*args, **kwargs):
with self:
return func(*args, **kwargs)
return decorate_no_grad
However there is an additional functionality of torch.set_grad_enabled
over torch.no_grad
when used in a with
-statement which lets you control to switch on or off gradient computation:
>>> x = torch.tensor([1], requires_grad=True)
>>> is_train = False
>>> with torch.set_grad_enabled(is_train):
... y = x * 2
>>> y.requires_grad
https://pytorch.org/docs/stable/_modules/torch/autograd/grad_mode.html
add a comment |
Actually no, there no difference in the way used in the question. When you take a look at the source code of no_grad
. You see that it is actually using torch.set_grad_enabled
to archive this behaviour:
class no_grad(object):
r"""Context-manager that disabled gradient calculation.
Disabling gradient calculation is useful for inference, when you are sure
that you will not call :meth:`Tensor.backward()`. It will reduce memory
consumption for computations that would otherwise have `requires_grad=True`.
In this mode, the result of every computation will have
`requires_grad=False`, even when the inputs have `requires_grad=True`.
Also functions as a decorator.
Example::
>>> x = torch.tensor([1], requires_grad=True)
>>> with torch.no_grad():
... y = x * 2
>>> y.requires_grad
False
>>> @torch.no_grad()
... def doubler(x):
... return x * 2
>>> z = doubler(x)
>>> z.requires_grad
False
"""
def __init__(self):
self.prev = torch.is_grad_enabled()
def __enter__(self):
torch._C.set_grad_enabled(False)
def __exit__(self, *args):
torch.set_grad_enabled(self.prev)
return False
def __call__(self, func):
@functools.wraps(func)
def decorate_no_grad(*args, **kwargs):
with self:
return func(*args, **kwargs)
return decorate_no_grad
However there is an additional functionality of torch.set_grad_enabled
over torch.no_grad
when used in a with
-statement which lets you control to switch on or off gradient computation:
>>> x = torch.tensor([1], requires_grad=True)
>>> is_train = False
>>> with torch.set_grad_enabled(is_train):
... y = x * 2
>>> y.requires_grad
https://pytorch.org/docs/stable/_modules/torch/autograd/grad_mode.html
Actually no, there no difference in the way used in the question. When you take a look at the source code of no_grad
. You see that it is actually using torch.set_grad_enabled
to archive this behaviour:
class no_grad(object):
r"""Context-manager that disabled gradient calculation.
Disabling gradient calculation is useful for inference, when you are sure
that you will not call :meth:`Tensor.backward()`. It will reduce memory
consumption for computations that would otherwise have `requires_grad=True`.
In this mode, the result of every computation will have
`requires_grad=False`, even when the inputs have `requires_grad=True`.
Also functions as a decorator.
Example::
>>> x = torch.tensor([1], requires_grad=True)
>>> with torch.no_grad():
... y = x * 2
>>> y.requires_grad
False
>>> @torch.no_grad()
... def doubler(x):
... return x * 2
>>> z = doubler(x)
>>> z.requires_grad
False
"""
def __init__(self):
self.prev = torch.is_grad_enabled()
def __enter__(self):
torch._C.set_grad_enabled(False)
def __exit__(self, *args):
torch.set_grad_enabled(self.prev)
return False
def __call__(self, func):
@functools.wraps(func)
def decorate_no_grad(*args, **kwargs):
with self:
return func(*args, **kwargs)
return decorate_no_grad
However there is an additional functionality of torch.set_grad_enabled
over torch.no_grad
when used in a with
-statement which lets you control to switch on or off gradient computation:
>>> x = torch.tensor([1], requires_grad=True)
>>> is_train = False
>>> with torch.set_grad_enabled(is_train):
... y = x * 2
>>> y.requires_grad
https://pytorch.org/docs/stable/_modules/torch/autograd/grad_mode.html
edited Nov 23 '18 at 14:45
answered Nov 23 '18 at 13:30


blue-phoenoxblue-phoenox
4,216101643
4,216101643
add a comment |
add a comment |
Thanks for contributing an answer to Stack Overflow!
- Please be sure to answer the question. Provide details and share your research!
But avoid …
- Asking for help, clarification, or responding to other answers.
- Making statements based on opinion; back them up with references or personal experience.
To learn more, see our tips on writing great answers.
Sign up or log in
StackExchange.ready(function () {
StackExchange.helpers.onClickDraftSave('#login-link');
});
Sign up using Google
Sign up using Facebook
Sign up using Email and Password
Post as a guest
Required, but never shown
StackExchange.ready(
function () {
StackExchange.openid.initPostLogin('.new-post-login', 'https%3a%2f%2fstackoverflow.com%2fquestions%2f53447345%2fpytorch-set-grad-enabledfalse-vs-with-no-grad%23new-answer', 'question_page');
}
);
Post as a guest
Required, but never shown
Sign up or log in
StackExchange.ready(function () {
StackExchange.helpers.onClickDraftSave('#login-link');
});
Sign up using Google
Sign up using Facebook
Sign up using Email and Password
Post as a guest
Required, but never shown
Sign up or log in
StackExchange.ready(function () {
StackExchange.helpers.onClickDraftSave('#login-link');
});
Sign up using Google
Sign up using Facebook
Sign up using Email and Password
Post as a guest
Required, but never shown
Sign up or log in
StackExchange.ready(function () {
StackExchange.helpers.onClickDraftSave('#login-link');
});
Sign up using Google
Sign up using Facebook
Sign up using Email and Password
Sign up using Google
Sign up using Facebook
Sign up using Email and Password
Post as a guest
Required, but never shown
Required, but never shown
Required, but never shown
Required, but never shown
Required, but never shown
Required, but never shown
Required, but never shown
Required, but never shown
Required, but never shown
c3u6viKRodW3AvA,L,XamDII4a2oIVbpZUG1W,9A5pkNqI 4ep w,Q,w
Related: Save and restore autograd enabled state
– Tom Hale
Nov 23 '18 at 13:15